Peer reviewed papers supporting Synthetic Users
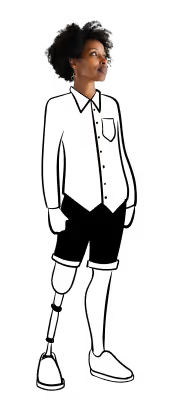
Simulating the Human in HCD with ChatGPT: Redesigning Interaction Design with AI
Authors: Albrecht Schmidt, Passant Elagroudy, Fiona Draxler, Frauke Kreuter, Robin Welsch
Published: January-February 2024 in Interactions Magazine
Research Question: How can large language models (LLMs) and generative AI be used to augment or replace human input in human-centered design (HCD) processes?
Key Methodologies
- Exploration of LLM capabilities in various HCD stages
- Analysis of potential applications and limitations of AI in HCD
- Consideration of ethical implications
Primary Findings
- LLMs can support HCD in multiple ways, including stakeholder identification, persona creation, and prototype generation
- AI can enhance efficiency and scalability in HCD processes
- Transparency about AI usage in HCD is crucial
- AI should complement rather than fully replace human involvement in HCD
Relevance to Synthetic Users
AI-generated personas and stakeholders: The paper discusses using LLMs to create personas and identify stakeholders, which directly relates to the concept of Synthetic Users. For example, the authors state: "AI can help researchers auto-generate their personas while embedding deeper backgrounds about marginalized groups, such as users with accessibility needs."
Simulated user responses: The authors explore the possibility of using LLMs to simulate user responses in surveys and focus groups, which aligns with the core idea of Synthetic Users. They note: "LLMs can be used as a simulation of the participants of a survey. This is the most controversial usage in our view."
AI-augmented evaluation processes: The paper discusses using AI to enhance evaluation processes in HCD, including simulating a wide range of personas and usage scenarios. This relates to the potential application of Synthetic Users in preliminary testing phases.
Supporting Evidence
The authors suggest that LLMs can be used to generate diverse personas and simulate user responses, potentially overcoming limitations in accessing certain user groups. They state: "Most often, however, these same people have shared and discussed their experiences in Internet forums; hence, we may be able to retrieve their views and opinions through the use of LLMs."
Impact On Synthetic Users: This supports the potential of Synthetic Users to provide insights from diverse backgrounds and hard-to-reach populations, addressing a key aspect of the concept.
The paper discusses the potential for LLMs to speed up and scale various aspects of the HCD process, including stakeholder identification, persona creation, and prototype generation.
Impact On Synthetic Users: This aligns with the proposed benefits of Synthetic Users in scaling research efforts and exploring hypothetical scenarios more efficiently.
Contradictory Evidence
The authors emphasize the importance of transparency when using AI in HCD processes, stating: "The approach taken, however, should be made transparent to the customer or stakeholder in a commercial setting or the reader of a paper in the scientific space."
Impact On Synthetic Users: This highlights potential ethical concerns with Synthetic Users if not clearly disclosed, potentially limiting their acceptance or applicability in certain research contexts.
The paper acknowledges limitations in AI's ability to capture certain aspects of human experience, noting: "In some fields of HCI like user interface design, empathetic computing, or social VR, we create experiences that support intuitive use, enhance our emotional palette, or create bonds between people. If current AI is devoid of emulating these aspects of human experience, then we might consider refraining from using AI in HCD altogether."
Impact On Synthetic Users: This suggests that Synthetic Users may have significant limitations in accurately representing human emotional and social experiences, potentially reducing their effectiveness in certain research areas.
Gaps and Opportunities
The paper does not provide empirical evidence comparing the accuracy of AI-generated responses to those of real human participants across different demographics.
Potential Solution: Conduct comparative studies between AI-generated responses and responses from diverse human participants to validate the accuracy and representativeness of Synthetic Users.
The authors do not deeply explore the potential for fine-tuning or specialized training of LLMs for specific demographic groups or user contexts.
Potential Solution: Investigate methods for creating more targeted and accurate Synthetic Users through specialized AI training on demographic-specific data sets.
Ethical Considerations
The paper raises concerns about the reproduction of biases in AI-generated outputs, stating: "As with many other AI systems, LLMs have been shown to reproduce human biases and stereotypes present in the training data."
Relevance To Synthetic Users: This highlights the need for careful consideration and mitigation of biases when developing and applying Synthetic Users to ensure fair and accurate representation of diverse groups.
The authors emphasize the importance of transparency in AI usage, which is crucial for maintaining ethical standards in research.
Relevance To Synthetic Users: Implementation of Synthetic Users would require clear disclosure and explanation of their nature and limitations to maintain research integrity and participant trust.
Practical Applications
The paper suggests using AI-generated personas and simulated responses in early stages of design and ideation.
Comparison To Traditional Methods: This could allow for more rapid and diverse idea generation compared to traditional methods, potentially leading to more innovative designs. However, it may lack the nuanced insights that come from direct human interaction.
The authors propose using AI to enhance prototype evaluation by simulating a wide range of user interactions.
Comparison To Traditional Methods: This could provide a broader scope of feedback more quickly than traditional user testing, but may miss subtle usability issues that real users might encounter.
Limitations and Challenges
The paper notes the potential for AI hallucinations and speculative responses when prompts are vague.
Potential Mitigation: Develop rigorous prompting strategies and validation methods to ensure the reliability of Synthetic User responses.
The authors raise concerns about the dilution of human perspective as AI-generated content increases in prevalence.
Potential Mitigation: Implement safeguards to ensure Synthetic Users are trained on verified human-generated data and regularly updated to reflect current human perspectives.
Future Research Directions
Investigate the long-term impacts of AI-augmented HCD on design outcomes and user satisfaction.
Rationale: Understanding these impacts is crucial for determining the appropriate balance between AI and human involvement in the design process.
Explore methods for combining AI-generated insights with traditional user research to create more comprehensive and accurate user representations.
Rationale: This could leverage the strengths of both approaches to enhance the overall quality of user research and design outcomes.
Accuracy of Demographic Mimicry
Findings: The paper does not provide specific findings on the accuracy of AI in mimicking different demographics. However, it suggests that LLMs can potentially capture diverse perspectives due to their training on vast amounts of data, including personal experience reports.
Implications for Synthetic Users: While the potential for accurate demographic mimicry is suggested, the lack of empirical evidence highlights the need for extensive validation of Synthetic Users across different demographic groups before they can be reliably used in research contexts.
Overall Assessment
The paper presents a balanced view of the potential for AI, particularly LLMs, to augment and potentially replace certain aspects of human involvement in HCD processes. While it highlights significant opportunities for efficiency and scalability, it also emphasizes the importance of maintaining human involvement and ethical considerations in AI application.
Relation to Synthetic Users: supports
The paper generally supports the concept of Synthetic Users by exploring various ways AI can simulate human input in design processes. However, it also highlights important limitations and ethical considerations that must be addressed for successful implementation.
Suggested Refinements
Refinement: Develop clear guidelines for when and how to use AI-generated personas and responses in HCD processes.
Justification: This would help address the ethical concerns raised in the paper and ensure appropriate application of Synthetic Users.
Refinement: Conduct empirical studies to validate the accuracy and representativeness of AI-generated responses across diverse demographic groups.
Justification: This would address the gap in evidence regarding the accuracy of demographic mimicry, which is crucial for the validity of Synthetic Users.
Embracing naturalistic paradigms: substituting GPT predictions for human judgments
Authors: Xuan Yang, Christian O'Reilly, Svetlana V. Shinkareva
Published: June 22, 2024 (preprint) in bioRxiv preprint
Research Question: Can GPT language models reliably substitute for human judgments in rating the affective content of narratives?
Key Methodologies
- Comparison of GPT-4 ratings to human ratings for valence and arousal in narrative segments
- Correlation of GPT and human ratings with fMRI data from participants listening to narratives
- Simulation to determine number of human raters needed to outperform GPT
Primary Findings
- GPT ratings highly correlated with human ratings (mean correlation coefficient = 0.81)
- GPT outperformed single human raters and lexical-level affective ratings
- Brain regions identified using GPT ratings were remarkably similar to those identified using human ratings
- 3-5 human raters were needed on average to outperform GPT ratings
Relevance to Synthetic Users
AI-generated responses as proxies for human judgments: The study demonstrates that GPT can produce affective ratings highly correlated with human judgments, supporting the core premise of Synthetic Users that AI can generate human-like responses.
Scalability and efficiency of AI-based research methods: The paper highlights the time and cost savings of using GPT compared to traditional human participant studies, aligning with the Synthetic Users concept's proposed benefits for scaling research efforts.
Accuracy of AI in mimicking human responses: The study provides evidence that GPT can accurately mimic human affective judgments, addressing a key question in the Synthetic Users concept about the ability of AI to represent human perspectives.
Supporting Evidence
GPT ratings were highly correlated with human ratings (mean correlation coefficient = 0.81) and closely reproduced the U-shape relationship between arousal and valence observed in human ratings.
Impact On Synthetic Users: This strong correlation supports the potential of using AI-generated responses as reliable proxies for human judgments in certain tasks, a key premise of the Synthetic Users concept.
GPT ratings outperformed single human raters and even small groups of human raters (up to about five), suggesting high reliability and consistency.
Impact On Synthetic Users: This finding strengthens the case for using AI-generated responses in place of small numbers of human participants, potentially allowing for more efficient and scalable research methodologies.
Brain regions identified using GPT ratings in fMRI analysis were remarkably similar to those identified using human ratings, indicating biological validity of the AI-generated responses.
Impact On Synthetic Users: This neurobiological evidence adds weight to the argument that AI-generated responses can serve as meaningful proxies for human judgments, even at the level of brain activity.
Contradictory Evidence
GPT made some alignment errors when parsing and rating narrative segments, indicating imperfect performance in complex, multifaceted tasks.
Impact On Synthetic Users: This suggests that there may be limitations to the accuracy and reliability of AI-generated responses in more complex scenarios, potentially requiring human oversight or refinement of prompting strategies.
The study focused on a specific task (affective ratings of narratives) and used a particular AI model (GPT-4), limiting generalizability to other tasks or AI systems.
Impact On Synthetic Users: This narrow focus raises questions about how well the findings would translate to other types of judgments or user research scenarios proposed in the Synthetic Users concept.
Gaps and Opportunities
The study did not explore demographic-specific responses or attempt to generate responses for diverse user personas.
Potential Solution: Future research could investigate GPT's ability to generate affective ratings that mimic specific demographic groups, comparing these to human ratings from those groups.
The paper did not address the ethical implications of substituting AI judgments for human participants.
Potential Solution: A follow-up study could explore the ethical considerations of using AI-generated responses in research, including potential biases and the impact on human participation in studies.
Ethical Considerations
The paper does not directly address the ethical implications of substituting AI judgments for human participants in research.
Relevance To Synthetic Users: This is a critical consideration for the Synthetic Users concept, as it raises questions about the ethical implications of replacing human participants with AI-generated responses in various research contexts.
The study demonstrates that GPT can produce human-like affective judgments, which could have implications for privacy and consent in future research using AI-generated responses.
Relevance To Synthetic Users: As Synthetic Users aims to represent diverse demographics, careful consideration must be given to how personal data and characteristics are used to generate AI responses, and whether this constitutes a form of synthetic data that requires ethical oversight.
Practical Applications
Using GPT or similar AI models to generate affective ratings for narrative content in media research, content creation, or user experience design.
Comparison To Traditional Methods: This approach could be significantly faster and more cost-effective than recruiting human participants, while still providing reliable results that correlate with brain activity.
Employing AI-generated ratings as a preliminary step in research design, helping to identify potentially interesting stimuli or hypotheses before involving human participants.
Comparison To Traditional Methods: This could streamline the research process and potentially reduce the number of human participants needed, saving time and resources while still maintaining scientific validity.
Limitations and Challenges
The study focused on a specific task (affective ratings) and stimulus type (narratives), limiting generalizability to other domains of user research.
Potential Mitigation: Conduct similar studies across a wider range of tasks and stimuli to establish the broader applicability of AI-generated responses in user research.
The paper does not address potential biases in the AI model's training data or output, which could impact the representativeness of its judgments.
Potential Mitigation: Investigate and quantify potential biases in AI-generated responses, comparing them to known biases in human participant samples.
Future Research Directions
Explore GPT's ability to generate responses that mimic specific demographic groups or user personas.
Rationale: This would directly address the core question of the Synthetic Users concept regarding AI's ability to accurately represent diverse user groups.
Investigate the potential for fine-tuning AI models on specific demographic data to improve their ability to generate representative responses.
Rationale: This could enhance the accuracy and utility of AI-generated responses in representing diverse user groups, a key goal of the Synthetic Users concept.
Conduct studies comparing AI-generated responses to human responses across a wider range of research tasks and methodologies beyond affective ratings.
Rationale: This would help establish the broader applicability and limitations of using AI-generated responses in user research and market analysis.
Accuracy of Demographic Mimicry
Findings: The study did not directly address demographic-specific mimicry, focusing instead on overall correlation with aggregate human judgments. However, the high correlation between GPT and human ratings suggests potential for accurate mimicry.
Implications for Synthetic Users: While promising, further research is needed to determine if AI can accurately mimic responses from specific demographic groups. The study's methodology could be adapted to investigate this by comparing AI-generated responses to those from specific demographic subgroups.
Overall Assessment
The study provides strong evidence for the ability of GPT to generate human-like affective judgments in narrative contexts, with high correlation to human ratings and similar patterns of associated brain activity. These findings support the potential of using AI-generated responses as proxies for human judgments in certain research contexts, aligning with key aspects of the Synthetic Users concept.
Relation to Synthetic Users: supports
The paper demonstrates that AI can generate responses highly correlated with human judgments, outperforming individual human raters in some cases. This supports the core premise of Synthetic Users that AI could potentially serve as a proxy for human participants in certain research contexts. However, the study's focus on affective ratings of narratives limits its direct application to the broader goals of Synthetic Users in mimicking diverse demographic groups across various tasks.
Suggested Refinements
Refinement: Investigate GPT's ability to generate responses mimicking specific demographic groups or user personas.
Justification: This would directly address the Synthetic Users concept's goal of representing diverse user groups and help determine the limits of AI in accurately mimicking specific demographics.
Refinement: Expand the range of tasks and judgments beyond affective ratings to include other aspects of user research and market analysis.
Justification: This would help establish the broader applicability and limitations of using AI-generated responses as proxies for human judgments across various research contexts.
The Fill-Mask Association Test (FMAT): Measuring Propositions in Natural Language
Authors: Han-Wu-Shuang Bao
Published: In press (as of April 12, 2024) in Journal of Personality and Social Psychology
Research Question: How well can the Fill-Mask Association Test (FMAT) measure semantic associations in natural language?
Key Methodologies
- Use of BERT language models to compute semantic probabilities of words filling in masked blanks in designed queries
- Comparison of FMAT results to previous findings from human participants and other text analysis methods
- 15 studies examining factual associations, attitudes/biases, social stereotypes, and sociocultural changes over time
Primary Findings
- FMAT demonstrated good reliability and validity in measuring various psychological constructs
- Results replicated seminal findings previously obtained with human participants and other methods
- FMAT allowed for more fine-grained measurement of theoretical constructs by specifying relational information
- Findings support a propositional perspective on how semantic associations are represented in natural language
Relevance to Synthetic Users
Accuracy of AI language models in capturing human-like associations: The FMAT method demonstrates that BERT models can reliably capture semantic associations similar to those found in human cognition, suggesting potential for synthetic users to exhibit human-like patterns of thought.
Measurement of implicit attitudes and biases: FMAT's ability to measure implicit attitudes and biases indicates that synthetic users based on similar models might be able to simulate these subtle aspects of human cognition.
Representation of cultural and historical knowledge: The study's findings on sociocultural changes suggest that language models can encode cultural and historical information, which could inform the creation of synthetic users from different time periods or cultural backgrounds.
Supporting Evidence
The FMAT demonstrated high reliability and validity in predicting factual associations, such as gender distribution in occupations and names. For example, in Study 1A, FMAT gender scores of 50 occupations strongly correlated with actual percentages of male workers (r = .74, p < .001).
Impact On Synthetic Users: This suggests that synthetic users based on similar language models could accurately represent factual knowledge about demographic distributions in various domains.
Studies 2A-2D showed that FMAT could replicate classic findings on attitudes and biases, such as implicit preferences for flowers over insects or young over old people. The method was also able to distinguish between attitudinal and non-attitudinal associations.
Impact On Synthetic Users: This indicates that synthetic users might be capable of simulating both explicit and implicit attitudes, as well as differentiating between various types of associations, leading to more nuanced and realistic responses.
Studies 4A-4D demonstrated FMAT's ability to capture perceived sociocultural changes over time, such as declining gender and racial biases in occupational participation and increasing individualism in American culture.
Impact On Synthetic Users: This suggests that synthetic users could potentially be calibrated to represent perspectives from different historical periods or to simulate changing cultural attitudes over time.
Contradictory Evidence
The paper notes that FMAT effect sizes were often smaller than those found with human participants using methods like the Implicit Association Test (IAT). For example, in Study 2A, the FMAT effect size for flower-insect attitude was d = 0.37, compared to d = 1.35 for the IAT.
Impact On Synthetic Users: This suggests that synthetic users based on similar models might exhibit weaker or less extreme attitudes and biases compared to real humans, potentially leading to underestimation of the strength of human biases.
The author acknowledges that the exact demographic characteristics of the text producers for the training corpora are unlikely to be identified, although they are assumed to be primarily English speakers from Anglophone countries.
Impact On Synthetic Users: This limitation in demographic specificity of the training data could lead to synthetic users that are biased towards certain populations and may not accurately represent diverse demographic groups.
Gaps and Opportunities
The study focuses on English language models and primarily Anglophone perspectives. There is a need to extend the research to other languages and cultures.
Potential Solution: Future research could apply the FMAT method to BERT models trained on non-English corpora to create more culturally diverse synthetic users.
The paper does not explore individual differences or specific demographic subgroups beyond broad categories like gender and race.
Potential Solution: Further studies could investigate how well language models capture more fine-grained individual differences or represent specific demographic subgroups, informing the creation of more personalized synthetic users.
Ethical Considerations
The paper raises concerns about the potential for language models to inherit and perpetuate societal biases present in their training data.
Relevance To Synthetic Users: When creating synthetic users, researchers must be aware that these AI-generated personas may reflect and potentially amplify existing societal biases, requiring careful monitoring and mitigation strategies.
The author notes that some BERT models have been intentionally debiased, which could distort data that should reflect social reality for research purposes.
Relevance To Synthetic Users: This raises questions about whether synthetic users should be designed to reflect actual human biases for accurate representation, or if they should be debiased to promote more ethical outcomes.
Practical Applications
The FMAT method could be adapted to generate responses from synthetic users by using the most probable word completions for carefully designed queries.
Comparison To Traditional Methods: This approach would allow for more efficient and scalable collection of responses compared to traditional human participant recruitment, while still capturing nuanced associations and attitudes.
The ability of FMAT to capture perceived historical changes could be used to create synthetic users representing different time periods or to simulate changing attitudes over time.
Comparison To Traditional Methods: This would provide a unique capability to explore historical perspectives or project future trends, which is challenging to achieve with traditional research methods relying on contemporary human participants.
Limitations and Challenges
The study relies on pre-trained BERT models, which may not always reflect the most current societal attitudes or knowledge.
Potential Mitigation: Regular updating and fine-tuning of language models with more recent data could help keep synthetic users current and relevant.
The paper notes that a single BERT model often produces unreliable results, requiring aggregation across multiple models for robust findings.
Potential Mitigation: When implementing synthetic users, responses should be generated from an ensemble of models to ensure greater reliability and consistency.
Future Research Directions
Investigate how well the FMAT method and similar approaches can capture more complex psychological constructs beyond attitudes and stereotypes, such as personality traits or decision-making processes.
Rationale: This would expand the range of human characteristics that could be accurately simulated in synthetic users, increasing their realism and utility for various research applications.
Explore the potential for fine-tuning language models on specific demographic subgroups to create more targeted and accurate synthetic user profiles.
Rationale: This could address the current limitations in demographic specificity and enable the creation of synthetic users that more precisely represent particular population segments.
Accuracy of Demographic Mimicry
Findings: The study demonstrates that BERT models can accurately capture broad demographic trends, such as gender distributions in occupations and names (Studies 1A-1C). It also shows that these models can reflect cultural differences over time (Studies 4A-4D). However, the research does not delve into fine-grained demographic distinctions or individual-level accuracy.
Implications for Synthetic Users: While synthetic users based on these models might accurately represent broad demographic trends and cultural shifts, they may struggle to capture nuanced differences between individuals or specific subgroups within larger demographic categories. Careful validation would be needed to ensure synthetic users accurately represent the intended demographic profiles, especially for more specific or intersectional identities.
Overall Assessment
The Fill-Mask Association Test (FMAT) demonstrates that large language models can reliably capture human-like semantic associations, attitudes, and biases, as well as reflect cultural and historical knowledge. This suggests significant potential for creating synthetic users that exhibit realistic cognitive patterns and cultural awareness. However, limitations in demographic specificity, potential perpetuation of societal biases, and the need for model ensembles present challenges that must be addressed for effective implementation.
Relation to Synthetic Users: supports
The FMAT's success in replicating various psychological phenomena using language models provides strong support for the feasibility of synthetic users. The method's ability to capture subtle attitudes, cultural knowledge, and historical changes suggests that synthetic users could potentially provide rich, nuanced responses across a wide range of domains. However, the identified limitations and ethical considerations indicate that careful design and validation processes would be crucial for creating accurate and responsible synthetic user systems.
Suggested Refinements
Refinement: Develop methods to fine-tune language models on more specific demographic data to create more accurate and diverse synthetic user profiles.
Justification: This would address the current limitations in demographic specificity and allow for the creation of synthetic users that more precisely represent particular population segments or individuals.
Refinement: Incorporate explicit bias detection and mitigation techniques in the synthetic user generation process.
Justification: This would help address ethical concerns about perpetuating societal biases while maintaining the ability to accurately represent human cognition when necessary for research purposes.
Revealing Fine-Grained Values and Opinions in Large Language Models
Authors: Dustin Wright, Arnav Arora, Nadav Borenstein, Srishti Yadav, Serge Belongie, Isabelle Augenstein
Published: 2024 in arXiv preprint
Research Question: How do demographic-based prompts impact LLM survey responses, and what tropes do LLMs produce in response to political compass test propositions?
Key Methodologies
- Generated responses to Political Compass Test propositions using 6 LLMs with 420 prompt variations
- Analyzed closed-form and open-ended responses
- Developed method to extract "tropes" (recurring themes/motifs) from open-ended responses
- Compared responses across different demographic prompts
Primary Findings
- Demographic features in prompts significantly affect LLM responses on the Political Compass Test
- There are disparities between closed-form and open-ended responses
- Similar justifications (tropes) are repeatedly generated across models and prompts even with disparate stances
- Some models (e.g. OLMo) showed less variation in responses across demographic prompts
Relevance to Synthetic Users
Demographic Mimicry: The paper directly examines how LLMs respond when prompted with different demographic characteristics, which is central to the concept of Synthetic Users and their ability to represent diverse populations.
Response Consistency: The study's analysis of tropes reveals patterns in LLM responses across different prompts, which is relevant to understanding the reliability and consistency of Synthetic Users.
Model Variability: The paper's comparison of different LLMs shows that some models are more affected by demographic prompts than others, which has implications for selecting appropriate models for Synthetic Users.
Supporting Evidence
The study found significant effects of demographic prompts on LLM responses to the Political Compass Test, particularly for political orientation, gender, and economic class.
Impact On Synthetic Users: This suggests that LLMs can potentially mimic different demographic groups to some extent, supporting the feasibility of Synthetic Users.
The extraction of tropes revealed common justifications used across different models and prompts, even when the final stances differed.
Impact On Synthetic Users: This indicates that LLMs can generate coherent and consistent reasoning patterns, which is important for creating believable Synthetic Users.
Contradictory Evidence
The study found disparities between closed-form and open-ended responses, with models often giving more neutral or refusing to answer in open-ended formats.
Impact On Synthetic Users: This suggests that Synthetic Users may not consistently mimic human behavior across different response formats, potentially limiting their applicability.
Some models, like OLMo, showed little variation in responses across demographic prompts.
Impact On Synthetic Users: This indicates that not all LLMs are equally capable of mimicking different demographics, which could limit the diversity of Synthetic Users depending on the model used.
Gaps and Opportunities
The study focused on political opinions and values, but did not explore other aspects of user behavior or preferences.
Potential Solution: Future research could apply similar methodologies to analyze LLM responses in other domains relevant to user research, such as product preferences or user experience.
The paper did not compare LLM responses to actual human responses from different demographic groups.
Potential Solution: A follow-up study could administer the same Political Compass Test to human participants from various demographics and compare their responses to those generated by LLMs.
Ethical Considerations
The study reveals that LLMs can be steered to generate biased responses based on demographic prompts.
Relevance To Synthetic Users: This raises concerns about the potential for Synthetic Users to perpetuate or amplify societal biases if not carefully designed and monitored.
The paper shows that LLMs can generate coherent justifications for various political stances, even when those stances change based on prompts.
Relevance To Synthetic Users: This capability could be misused to generate artificial support for particular viewpoints, necessitating careful ethical guidelines for the use of Synthetic Users in research or public discourse.
Practical Applications
Using LLMs with demographic prompts to generate diverse perspectives on political or social issues.
Comparison To Traditional Methods: This could potentially provide a broader range of viewpoints more quickly and at lower cost than traditional survey methods, but may lack the nuance and authenticity of real human responses.
Analyzing tropes in LLM responses to understand common reasoning patterns associated with different demographics or viewpoints.
Comparison To Traditional Methods: This could offer insights into argumentation structures more efficiently than manual coding of human responses, but may miss important nuances or novel arguments that humans might provide.
Limitations and Challenges
The study found that LLM responses can vary significantly based on small changes in prompts or question formats.
Potential Mitigation: Developing more robust prompting techniques or aggregating responses across multiple prompts could help create more stable Synthetic Users.
The paper focused on English-language models and Western political concepts.
Potential Mitigation: Future research should explore the capabilities of multilingual models and consider non-Western cultural and political frameworks to create more globally representative Synthetic Users.
Future Research Directions
Investigate the long-term consistency of LLM-generated personas across multiple interactions or conversations.
Rationale: This would help determine if Synthetic Users can maintain coherent personalities and viewpoints over time, which is crucial for their use in extended user research scenarios.
Explore techniques to fine-tune LLMs on real demographic data to improve their mimicry of specific user groups.
Rationale: This could potentially increase the accuracy and authenticity of Synthetic Users, making them more representative of real demographic groups.
Accuracy of Demographic Mimicry
Findings: The study demonstrates that LLMs can produce different responses when prompted with various demographic characteristics, particularly for political orientation, gender, and economic class. However, the accuracy of these responses in mimicking real demographic groups was not directly assessed.
Implications for Synthetic Users: While the results suggest that LLMs have some capability to generate responses aligned with different demographics, further research is needed to validate the accuracy of this mimicry against real human data. The variation in model performance (e.g., OLMo's relative invariance to demographic prompts) also indicates that the choice of LLM is crucial for creating effective Synthetic Users.
Overall Assessment
The paper provides valuable insights into the behavior of LLMs when prompted with demographic information, revealing both capabilities and limitations relevant to the concept of Synthetic Users. While the study demonstrates that LLMs can generate varied responses based on demographic prompts and produce consistent reasoning patterns (tropes), it also highlights challenges such as response format disparities and varying model performance.
Relation to Synthetic Users: supports
The research generally supports the potential of Synthetic Users by showing that LLMs can generate diverse responses based on demographic prompts. However, it also reveals important limitations and challenges that need to be addressed for effective implementation.
Suggested Refinements
Refinement: Incorporate real human data for validation
Justification: Comparing LLM-generated responses to those of real demographic groups would provide crucial validation of the accuracy of Synthetic Users.
Refinement: Develop techniques for maintaining consistency across different response formats
Justification: Addressing the disparities between closed-form and open-ended responses would improve the reliability and versatility of Synthetic Users.
Applications of GPT in Political Science Research
Authors: Kyuwon Lee, Simone Paci, Jeongmin Park, Hye Young You, Sylvan Zheng
Published: Not specified (recent) in Not specified
Research Question: How can GPT be applied to streamline data collection and analysis processes in political science research?
Key Methodologies
- Using GPT to clean OCR errors from scanned historical documents
- Extracting participant information from semi-structured administrative meeting minutes
- Extracting source information from news articles
- Synthesizing data from multiple internet sources
Primary Findings
- GPT can effectively clean OCR errors and extract information from various unstructured data sources
- GPT's performance matches or exceeds human efforts in many data extraction tasks
- GPT can significantly reduce time and financial resources required for data management in political science research
- Integration of GPT can make comprehensive data collection and analysis more accessible to researchers with limited resources
Relevance to Synthetic Users
AI-assisted data processing: The paper demonstrates GPT's ability to process and extract information from various data sources, which is relevant to the potential use of LLMs in generating synthetic user responses. The accuracy and efficiency of GPT in these tasks suggest that LLMs could potentially generate realistic user data across different contexts.
Comparison to human performance: The paper compares GPT's performance to human efforts in data extraction tasks. This relates to the Synthetic Users concept by providing insights into how well AI models can mimic human-level performance in information processing tasks, which is crucial for generating realistic synthetic user responses.
Supporting Evidence
The paper demonstrates GPT's ability to clean OCR errors from historical documents with accuracy comparable to high-quality OCR tools like Google Vision.
Impact On Synthetic Users: This suggests that LLMs like GPT have a strong capacity for understanding and processing natural language, which is crucial for generating realistic synthetic user responses across various demographics.
GPT successfully extracted participant information from semi-structured administrative meeting minutes, performing complex tasks like identifying diverse position labels.
Impact On Synthetic Users: This indicates that LLMs can handle nuanced contextual information, which is important for generating synthetic user responses that accurately reflect specific roles or backgrounds.
Contradictory Evidence
The paper notes that GPT occasionally exhibits "laziness" and does not follow task instructions, requiring multiple runs or human validation.
Impact On Synthetic Users: This suggests that LLMs may not always produce consistent or reliable responses, which could be problematic when generating synthetic user data that needs to accurately represent specific demographics or user groups.
Gaps and Opportunities
The paper focuses on data extraction and cleaning tasks rather than generating human-like responses or simulating user behavior.
Potential Solution: Future research could explore GPT's ability to generate synthetic user responses based on the extracted and cleaned data, potentially creating more diverse and representative synthetic user profiles.
Ethical Considerations
The paper discusses the potential impact of GPT on student research assistant positions, which are critical for financial support and professional growth.
Relevance To Synthetic Users: This raises ethical questions about the broader impacts of using AI-generated synthetic users in research, potentially reducing opportunities for human involvement and learning in the research process.
Practical Applications
Using GPT to extract and synthesize information from multiple internet sources for collecting biographical data on political elites.
Comparison To Traditional Methods: This application demonstrates significant time and resource savings compared to manual data collection methods, while maintaining comparable or better accuracy. For synthetic users, this suggests that LLMs could efficiently generate diverse user profiles based on real-world data sources.
Limitations and Challenges
The paper notes that GPT's performance degrades as text length increases, even for documents within the context window.
Potential Mitigation: For synthetic users, this limitation could be addressed by carefully designing prompts and breaking down complex scenarios into smaller, manageable chunks to ensure consistent performance across various user profiles and scenarios.
Future Research Directions
Explore GPT's ability to generate synthetic user responses based on extracted and analyzed data from various sources.
Rationale: This would bridge the gap between the data processing capabilities demonstrated in the paper and the goal of creating realistic synthetic user profiles for research purposes.
Accuracy of Demographic Mimicry
Findings: The paper shows that GPT can accurately extract and process information from diverse sources, including historical documents, meeting minutes, and news articles. In some cases, GPT outperformed human coders in tasks like identifying biographical information about political elites.
Implications for Synthetic Users: These findings suggest that LLMs have the potential to accurately process and understand diverse information sources, which is crucial for generating synthetic user responses that reflect various demographics. However, the paper does not directly address the generation of human-like responses, so further research would be needed to confirm GPT's ability to accurately mimic specific demographic or user groups.
Overall Assessment
The paper demonstrates GPT's strong capabilities in processing and extracting information from diverse data sources in political science research. While it does not directly address the creation of synthetic users, the findings suggest that LLMs have significant potential for understanding and working with complex, real-world data across various contexts.
Relation to Synthetic Users: supports
The paper's findings support the potential of using LLMs like GPT for creating synthetic users by demonstrating their ability to accurately process and understand diverse information sources. This capability is crucial for generating realistic and demographically accurate synthetic user responses. However, the paper does not directly address response generation, so additional research would be needed to fully validate the Synthetic Users concept.
Suggested Refinements
Refinement: Extend the research to include generating synthetic user responses based on the extracted and analyzed data.
Justification: This would provide direct evidence of GPT's ability to create realistic synthetic user profiles and responses, bridging the gap between data processing and the Synthetic Users concept.
Refinement: Conduct studies comparing GPT-generated synthetic user responses to real human responses across various demographics and contexts.
Justification: This would help validate the accuracy and representativeness of synthetic users created using LLMs, addressing the central question of how well these models can mimic specific demographic or user groups.
Large Language Models as Simulated Economic Agents: What Can We Learn from Homo Silicus?
Authors: John J. Horton
Published: April 2023 in NBER Working Paper
Research Question: Can large language models (LLMs) be used as simulated economic agents to replicate and extend findings from behavioral economics experiments?
Key Methodologies
- Using GPT-3 to simulate responses to classic behavioral economics experiments
- Comparing LLM responses to original human experimental results
- Extending experiments by varying parameters and scenarios
Primary Findings
- LLMs can qualitatively replicate findings from human experiments in behavioral economics
- LLMs can be "endowed" with different characteristics to simulate diverse perspectives
- LLM experiments are extremely fast and low-cost compared to human experiments
- More advanced LLM models (e.g. GPT-3 davinci-003) perform better than less capable models
Relevance to Synthetic Users
AI-generated responses mimicking human behavior: The paper demonstrates that LLMs can produce responses similar to those of human participants in behavioral economics experiments, supporting the core premise of Synthetic Users.
Endowing AI with specific characteristics: The author shows that LLMs can be given different "personalities" or viewpoints, aligning with the Synthetic Users concept of creating diverse AI-generated personas.
Scaling research efforts: The paper highlights the speed and low cost of running experiments with LLMs, supporting the Synthetic Users' potential for scaling research beyond traditional limitations.
Supporting Evidence
The paper successfully replicates key findings from classic behavioral economics experiments using GPT-3, including results on social preferences, fairness judgments, and status quo bias.
Impact On Synthetic Users: This supports the idea that Synthetic Users could potentially provide valid insights in certain research contexts, particularly in areas related to decision-making and economic behavior.
The author demonstrates that LLMs can be "endowed" with different political views or preferences, affecting their responses in predictable ways.
Impact On Synthetic Users: This suggests that Synthetic Users could potentially be customized to represent specific demographic or ideological groups, enhancing their utility in diverse research scenarios.
Contradictory Evidence
The paper notes that less capable LLM models (e.g., GPT-3 ada, babbage, and curie) often fail to change their responses based on endowed characteristics or framing.
Impact On Synthetic Users: This highlights that the effectiveness of Synthetic Users may be highly dependent on the specific AI model used, potentially limiting widespread application.
The author acknowledges that LLMs may have "memorized" certain experimental results or theories, potentially biasing their responses.
Impact On Synthetic Users: This raises concerns about the authenticity of Synthetic Users' responses and whether they truly represent novel insights or merely regurgitate existing knowledge.
Gaps and Opportunities
The paper focuses primarily on replicating existing experiments rather than exploring entirely new research questions.
Potential Solution: Future research could use LLMs to generate hypotheses or explore novel scenarios that would be difficult or impossible to test with human participants.
The study does not directly compare LLM responses to a contemporary human sample, relying instead on historical experimental results.
Potential Solution: Conduct parallel experiments with both LLMs and human participants to directly assess the accuracy of AI-generated responses.
Ethical Considerations
The paper raises concerns about the "performativity" problem, where LLMs might simply recite learned theories rather than provide genuine insights.
Relevance To Synthetic Users: This highlights the need for careful validation of Synthetic Users' responses to ensure they are not merely reproducing existing knowledge but providing authentic perspectives.
The author notes that using LLMs for experiments sidesteps human subjects-related ethical concerns.
Relevance To Synthetic Users: While this may simplify some research processes, it also raises questions about the ethics of simulating human perspectives and the potential for misuse or misrepresentation of AI-generated insights.
Practical Applications
The paper suggests using LLM experiments to pilot studies and explore parameter spaces before conducting human experiments.
Comparison To Traditional Methods: This approach could significantly reduce costs and time compared to traditional piloting methods, allowing researchers to refine their hypotheses and experimental designs more efficiently.
The author demonstrates how LLMs can be used to quickly test variations of experimental scenarios, such as different price points or political framings.
Comparison To Traditional Methods: This allows for much more extensive exploration of experimental conditions than would be feasible with human participants, potentially uncovering nuanced effects or unexpected relationships.
Limitations and Challenges
The paper notes that LLMs may have biases or inaccuracies in their training data, potentially leading to skewed or unrealistic responses.
Potential Mitigation: Researchers could cross-validate LLM responses with human data and continuously update models with high-quality, diverse training data.
The author acknowledges that LLMs may not always apply their "knowledge" consistently, similar to students who have crammed for an exam.
Potential Mitigation: Develop more sophisticated prompting techniques or fine-tuning methods to improve the consistency and applicability of LLM knowledge.
Future Research Directions
Investigate the potential for using LLMs to simulate specific types of economic agents, such as firms or policymakers.
Rationale: This could provide insights into complex economic systems and decision-making processes that are difficult to study with traditional methods.
Explore the use of LLMs in generating hypothetical scenarios or counterfactuals for economic policy analysis.
Rationale: This could help policymakers and researchers anticipate potential outcomes of different policy choices without real-world implementation risks.
Accuracy of Demographic Mimicry
Findings: The paper demonstrates that GPT-3 can produce responses that qualitatively match human behavior in several economic experiments when given appropriate prompts or "endowments." However, the author notes that this accuracy varies depending on the specific model used and the complexity of the task.
Implications for Synthetic Users: While the results are promising for certain applications, they also highlight the need for careful validation and potential limitations in accurately representing specific demographic groups. The paper does not provide direct evidence of LLMs' ability to mimic detailed demographic characteristics beyond broad ideological or political leanings.
Overall Assessment
The paper provides compelling evidence that large language models can effectively simulate human-like responses in certain behavioral economics experiments. It demonstrates the potential for using AI-generated agents as a tool for exploring economic behavior and decision-making. However, it also highlights important limitations and ethical considerations that must be addressed for responsible implementation.
Relation to Synthetic Users: supports
The paper largely supports the concept of Synthetic Users by showing that AI models can produce responses similar to human participants in specific contexts. It demonstrates the potential for using AI to simulate diverse perspectives and explore research questions efficiently. However, it also raises important caveats about the accuracy and authenticity of AI-generated responses.
Suggested Refinements
Refinement: Develop more robust methods for validating the accuracy of AI-generated responses across diverse demographic groups.
Justification: While the paper shows promise in simulating broad ideological perspectives, more work is needed to ensure Synthetic Users can accurately represent specific demographic characteristics.
Refinement: Investigate techniques to mitigate the risk of AI models simply reciting learned information rather than providing genuine insights.
Justification: Addressing the "performativity" problem is crucial for ensuring the authenticity and value of Synthetic Users in research contexts.
Virtual Personas for Language Models via an Anthology of Backstories
Authors: Suhong Moon, Marwa Abdulhai, Minwoo Kang, Joseph Suh, Widyadewi Soedarmadji, Eran Kohen Behar, David M. Chan
Published: July 9, 2024 in arXiv preprint
Research Question: How can large language models (LLMs) be conditioned to represent particular virtual personas that are representative, consistent, and diverse?
Key Methodologies
- Generating an "Anthology" of open-ended life narratives (backstories) using LLMs
- Conditioning LLMs with these backstories to create virtual personas
- Matching virtual personas to target human demographics
- Comparing responses of backstory-conditioned LLMs to human survey responses
Primary Findings
- Anthology method improved matching of human response distributions by up to 18%
- Consistency metrics improved by up to 27%
- Backstory-conditioned LLMs better represented diverse sub-populations
- Matching virtual personas to target demographics further enhanced approximation of human responses
Relevance to Synthetic Users
AI-generated personas: The paper's Anthology method uses LLMs to generate backstories, which are then used to condition LLMs to act as virtual personas. This directly aligns with the Synthetic Users concept of using AI to create simulated user personas.
Demographic representation: The paper focuses on creating virtual personas that match specific demographic profiles, which is a key aspect of the Synthetic Users concept for representing diverse user groups.
Survey response simulation: The research evaluates the ability of virtual personas to approximate human responses in large-scale surveys, which is a potential application of Synthetic Users in market research and user studies.
Supporting Evidence
The paper demonstrates improved performance in matching human response distributions: "Anthology achieves up to 18% improvement in matching the response distributions of human respondents and 27% improvement in consistency metrics."
Impact On Synthetic Users: This suggests that the Anthology method could enhance the accuracy of Synthetic Users in representing real human responses, particularly in survey-like scenarios.
The research shows better representation of diverse sub-populations: "We show superior conditioning to personas reflecting users from under-represented groups, with improvements of up to 18% in terms Wasserstein Distance and 27% in consistency."
Impact On Synthetic Users: This indicates that the Anthology approach could help address one of the key challenges in Synthetic Users - accurately representing diverse demographic groups.
Contradictory Evidence
The paper acknowledges limitations in fully simulating individual users: "We do not suggest that LLMs can fully simulate a given human user merely by using a user's backstory as a prompt prefix."
Impact On Synthetic Users: This highlights that while the Anthology method improves persona simulation, it still falls short of perfectly replicating individual human responses, which is a key consideration for the Synthetic Users concept.
The authors note potential biases in LLM-generated content: "There is an ongoing concern regarding the ethical use of virtual personas, especially regarding privacy, consent, and the potential for misuse in scenarios like deep fakes or manipulation in political and social spheres."
Impact On Synthetic Users: This raises ethical concerns about the use of Synthetic Users, particularly in sensitive contexts or where the AI-generated responses might be mistaken for real human opinions.
Gaps and Opportunities
The paper focuses primarily on survey responses and does not explore more complex user behaviors or interactions.
Potential Solution: Future research could extend the Anthology method to simulate more dynamic user interactions, such as product usage scenarios or customer service conversations.
The study is limited to text-based responses and does not consider multimodal data.
Potential Solution: Incorporating multimodal data (e.g., images, audio) into backstory generation and persona conditioning could create more comprehensive Synthetic Users.
Ethical Considerations
The paper raises concerns about privacy and consent in using AI-generated personas: "There is an ongoing concern regarding the ethical use of virtual personas, especially regarding privacy, consent, and the potential for misuse in scenarios like deep fakes or manipulation in political and social spheres."
Relevance To Synthetic Users: This highlights the need for clear ethical guidelines and transparency in the use of Synthetic Users, especially when they are used to represent real demographic groups or influence decision-making.
The authors discuss the potential for perpetuating biases: "If the training data is skewed or non-representative, the resulting personas may inadvertently perpetuate these biases."
Relevance To Synthetic Users: This emphasizes the importance of using diverse and representative data in training Synthetic Users to avoid reinforcing societal biases or misrepresenting certain groups.
Practical Applications
The paper demonstrates the use of virtual personas in approximating large-scale human studies, specifically the Pew Research Center's American Trends Panel (ATP) surveys.
Comparison To Traditional Methods: This approach could potentially reduce costs and time associated with recruiting large, diverse samples of human participants. It also allows for rapid iteration and testing of survey questions before deploying to real respondents.
The Anthology method could be used to generate diverse user personas for product testing and market research.
Comparison To Traditional Methods: This could allow companies to quickly simulate responses from a wide range of demographic groups without the need for extensive recruitment, potentially leading to more inclusive product design and marketing strategies.
Limitations and Challenges
The paper notes that "the computational cost associated with training and deploying state-of-the-art LLMs conditioned with detailed backstories is substantial, which may limit the scalability of this approach for widespread practical applications."
Potential Mitigation: Future research could focus on optimizing the Anthology method for efficiency, possibly through techniques like model distillation or more targeted backstory generation.
The authors acknowledge that "While backstories provide a rich context for generating personas, the current models may not consistently apply this context across different types of queries or interactions, leading to variability in persona consistency."
Potential Mitigation: Developing improved methods for maintaining persona consistency across varied interactions and contexts could enhance the reliability of Synthetic Users.
Future Research Directions
Extending the Anthology method to more complex, interactive scenarios beyond survey responses.
Rationale: This would test the limits of virtual personas in simulating real-world user behaviors and interactions, potentially expanding the applications of Synthetic Users.
Investigating the long-term stability and consistency of virtual personas across multiple interactions or over time.
Rationale: This would address concerns about the reliability of Synthetic Users in longitudinal studies or repeated interactions.
Accuracy of Demographic Mimicry
Findings: The paper reports improvements in matching human response distributions (up to 18%) and consistency metrics (up to 27%) when using the Anthology method. It also demonstrates better performance in representing under-represented groups.
Implications for Synthetic Users: These findings suggest that the Anthology method could significantly enhance the accuracy of Synthetic Users in mimicking specific demographic groups, particularly in survey-like contexts. However, the improvements, while substantial, still indicate a gap between virtual personas and real human responses, suggesting that further refinement is needed for perfect demographic mimicry.
Overall Assessment
The paper presents a promising approach for creating more representative and consistent virtual personas using LLMs, demonstrating improvements in matching human response distributions and representing diverse demographics. However, it also acknowledges limitations in fully simulating individual users and raises important ethical considerations.
Relation to Synthetic Users: supports
The Anthology method aligns closely with the goals of Synthetic Users, providing a concrete approach for generating diverse AI personas and demonstrating their potential in approximating human responses. The improvements in demographic representation and response consistency support the feasibility of using AI-generated personas in research contexts. However, the acknowledged limitations and ethical concerns highlight the need for careful implementation and further refinement of the Synthetic Users concept.
Suggested Refinements
Refinement: Incorporate more dynamic and interactive scenarios to test the limits of virtual persona consistency and adaptability.
Justification: This would address the current limitation of focusing primarily on survey responses and help determine the viability of Synthetic Users in more complex user research contexts.
Refinement: Develop robust ethical guidelines and transparency measures for the use of virtual personas in research and decision-making contexts.
Justification: This would address the ethical concerns raised in the paper and help establish trust in the use of Synthetic Users, particularly when representing real demographic groups.
Using Large Language Models to Simulate Multiple Humans and Replicate Human Subject Studies
Authors: Gati Aher, Rosa I. Arriaga, Adam Tauman Kalai
Published: 2023 in Proceedings of the 40th International Conference on Machine Learning
Research Question: To what extent can large language models (LLMs) simulate human behavior in experimental settings across diverse populations?
Key Methodologies
- Introducing Turing Experiments (TEs) to evaluate LLMs' ability to simulate human behavior
- Using prompts to generate synthetic experimental records from LLMs
- Replicating classic experiments: Ultimatum Game, Garden Path Sentences, Milgram Shock Experiment, Wisdom of Crowds
- Comparing LLM-generated results to human study findings
Primary Findings
- LLMs can replicate some human behavioral patterns in experimental settings
- Larger models generally produced more human-like responses
- Gender differences were observed in LLM-generated responses
- A "hyper-accuracy distortion" was found in newer, more aligned LLMs for general knowledge questions
Relevance to Synthetic Users
AI-generated diverse personas: The paper demonstrates LLMs' ability to generate responses for diverse "participants" by varying names and gender titles, aligning with the Synthetic Users concept of creating AI-generated personas representing various demographics.
Scalability of simulated responses: The study showcases the potential for LLMs to generate large numbers of simulated responses, addressing the Synthetic Users concept's goal of scaling research efforts beyond traditional recruitment limitations.
Accuracy of AI-generated responses: The paper directly addresses the central question of Synthetic Users by evaluating how accurately LLMs can mimic human responses across different experimental settings and demographics.
Supporting Evidence
The study found that larger language models (e.g., LM-5) were able to replicate known human behavioral patterns in experiments like the Ultimatum Game and Garden Path Sentences.
Impact On Synthetic Users: This suggests that advanced LLMs could potentially generate realistic synthetic user responses in certain contexts, supporting the feasibility of the Synthetic Users concept.
The paper demonstrated the ability to simulate gender differences in the Ultimatum Game TE, with the model showing a "chivalry effect" where men were more likely to accept unfair offers from women.
Impact On Synthetic Users: This indicates that LLMs can potentially capture and reproduce subtle demographic variations in behavior, which is crucial for creating diverse and realistic synthetic user personas.
Contradictory Evidence
The study uncovered a "hyper-accuracy distortion" in newer, more aligned LLMs when answering general knowledge questions in the Wisdom of Crowds TE.
Impact On Synthetic Users: This finding suggests that some LLMs may generate unrealistically accurate responses, which could limit their ability to faithfully represent typical user knowledge and behavior in certain scenarios.
The paper notes that LLMs have likely been exposed to descriptions of classic experiments like the Milgram Shock Experiment in their training data, potentially influencing their responses.
Impact On Synthetic Users: This highlights the challenge of ensuring that synthetic user responses are genuinely novel and not simply regurgitations of training data, which could limit their usefulness in research contexts.
Gaps and Opportunities
The study primarily focused on replicating existing experiments rather than generating novel scenarios or product concepts.
Potential Solution: Future research could explore LLMs' ability to generate synthetic user responses to entirely new scenarios or product ideas, more closely aligning with potential market research applications of Synthetic Users.
The paper did not extensively explore the impact of providing more detailed demographic information beyond names and gender titles.
Potential Solution: Further studies could investigate how providing richer demographic profiles (e.g., age, occupation, cultural background) affects the accuracy and diversity of LLM-generated responses.
Ethical Considerations
The paper raises ethical concerns about simulating harmful experiments like the Milgram Shock Experiment, even with AI-generated participants.
Relevance To Synthetic Users: This highlights the need for careful ethical guidelines in the use of Synthetic Users, particularly when exploring sensitive topics or potentially harmful scenarios.
The study acknowledges the risk of LLMs perpetuating biases present in their training data.
Relevance To Synthetic Users: This underscores the importance of critically examining and addressing potential biases in synthetic user responses to ensure they don't reinforce or exacerbate existing societal prejudices.
Practical Applications
Using LLMs to simulate diverse participant pools in preliminary research stages
Comparison To Traditional Methods: This approach could allow researchers to quickly and cost-effectively explore initial hypotheses with a large, diverse synthetic sample before investing in full-scale human participant studies.
Employing LLMs to generate synthetic responses in scenarios where human participation may be unethical or impractical
Comparison To Traditional Methods: This could enable exploration of sensitive topics or extreme situations that would be challenging or impossible to study with real human participants, while avoiding potential harm.
Limitations and Challenges
The study found that LLM performance varied across different tasks and model sizes, with smaller models often failing to accurately replicate human behavior.
Potential Mitigation: Careful selection and testing of LMs for specific research contexts, and potentially combining multiple models or approaches to improve overall accuracy.
The paper highlights the challenge of LLMs being sensitive to prompt wording and potentially exposed to relevant information in their training data.
Potential Mitigation: Developing robust prompting strategies and validation techniques to ensure generated responses are not overly influenced by specific phrasings or prior knowledge of experiments.
Future Research Directions
Investigating the impact of more detailed demographic profiles on LLM-generated responses
Rationale: This could help determine how much demographic information is needed to create accurate and diverse synthetic user personas.
Exploring LLMs' ability to generate responses for entirely novel scenarios or product concepts
Rationale: This would test the limits of LLMs in producing creative and realistic user feedback for unprecedented situations.
Developing methods to detect and mitigate unrealistic distortions like the "hyper-accuracy" effect
Rationale: This would improve the overall reliability and realism of synthetic user responses across various domains.
Accuracy of Demographic Mimicry
Findings: The study demonstrated that advanced LLMs could reproduce some demographic differences, such as gender effects in the Ultimatum Game. However, the accuracy varied across tasks and model sizes, with larger models generally performing better.
Implications for Synthetic Users: These findings suggest that while LLMs show promise in mimicking demographic variations, careful selection of models and extensive validation would be necessary to ensure accurate representation of diverse user groups in synthetic user research.
Overall Assessment
The paper provides compelling evidence that large language models can simulate human behavior in experimental settings with varying degrees of accuracy. While the results are promising for certain applications, challenges remain in ensuring consistent, unbiased, and realistic responses across diverse scenarios and demographics.
Relation to Synthetic Users: supports
The study demonstrates the potential of LLMs to generate diverse, human-like responses in experimental settings, which aligns with the core concept of Synthetic Users. However, it also highlights important limitations and ethical considerations that must be addressed for practical implementation.
Suggested Refinements
Refinement: Incorporate more detailed demographic profiles in LLM prompts
Justification: This could improve the accuracy and diversity of synthetic user responses, making them more representative of real-world populations.
Refinement: Develop techniques to mitigate unrealistic distortions like the "hyper-accuracy" effect
Justification: This would enhance the realism of synthetic user responses, particularly in knowledge-based tasks or market research scenarios.
Refinement: Establish rigorous ethical guidelines for the use of synthetic users in research
Justification: This would help address concerns about simulating harmful scenarios and potential biases in LLM-generated responses.
Estimating the Personality of White-Box Language Models
Authors: Saketh Reddy Karra, Son The Nguyen, Theja Tulabandhula
Published: May 10, 2023 in arXiv preprint
Research Question: Can the personality traits of large language models be quantified and potentially altered?
Key Methodologies
- Using the Big Five personality factors framework to assess language models
- Employing zero-shot learning classifiers to analyze model outputs
- Prompting language models with a personality assessment questionnaire
- Comparing personality traits of models to their training datasets
- Exploring methods to alter model personalities through fine-tuning
Primary Findings
- Language models exhibit quantifiable personality traits that can be measured
- Model personalities reflect traits of their training datasets to some degree
- Personality traits of models can be altered through fine-tuning, but with limited precision
- Different models show varied personality profiles across the Big Five factors
Relevance to Synthetic Users
Personality profiling of AI models: The paper's approach to quantifying personality traits in language models is directly relevant to assessing how accurately Synthetic Users could mimic human personality profiles. This methodology could be adapted to evaluate the personality authenticity of AI-generated personas.
Inheritance of traits from training data: The finding that models inherit personality traits from their training data suggests that careful curation of training datasets could potentially improve the accuracy of Synthetic Users in representing specific demographic groups.
Altering AI personality traits: The exploration of methods to alter model personalities through fine-tuning could inform techniques for adjusting Synthetic User personas to better match target demographics or user groups.
Supporting Evidence
The paper demonstrates that language models can be assessed for personality traits using established psychological frameworks like the Big Five factors. For example, the authors state: "Our work seeks to address this gap by exploring the personality traits of several large-scale language models designed for open-ended text generation and the datasets used for training them."
Impact On Synthetic Users: This suggests that Synthetic Users could potentially be evaluated and validated using similar personality assessment techniques, helping to ensure their responses align with intended demographic profiles.
The research shows that different language models exhibit varied personality profiles. As noted in the results: "We observe noticeable variations in trait distributions of text generated using different language models, as illustrated in Figures 5 and 6."
Impact On Synthetic Users: This variability across models indicates that careful selection and potential combination of different language models might be necessary to create Synthetic Users that accurately represent diverse personality types.
Contradictory Evidence
The paper highlights challenges in precisely controlling personality alterations through fine-tuning. The authors note: "However, we also observe noticeable changes in the scores of other personality traits, similar to Method 1, which is not desirable."
Impact On Synthetic Users: This suggests that creating Synthetic Users with very specific personality profiles may be difficult, potentially limiting the accuracy of demographic mimicry for highly targeted user groups.
Gaps and Opportunities
The paper focuses on white-box language models and does not explore more recent, advanced models like GPT-4 or instruction-tuned models.
Potential Solution: Future research could extend this methodology to assess personality traits in more advanced language models, which might offer improved capabilities for creating accurate Synthetic Users.
The study does not directly address how well the measured personality traits correspond to human perceptions of model outputs.
Potential Solution: Conducting human evaluation studies to compare perceived personality traits of model outputs with the measured traits could validate the approach and inform refinements for Synthetic Users.
Ethical Considerations
The paper raises concerns about biases and personality traits inherited by language models from their training data.
Relevance To Synthetic Users: This highlights the importance of carefully considering and mitigating potential biases in the creation of Synthetic Users to ensure fair and accurate representation of diverse groups.
The ability to alter model personalities through fine-tuning raises questions about the authenticity and potential manipulation of AI-generated personas.
Relevance To Synthetic Users: For Synthetic Users, there may be ethical concerns about creating artificial personas that are too perfectly tailored to research needs, potentially misrepresenting the true diversity of human perspectives.
Practical Applications
The methodology for assessing language model personalities could be adapted to validate and refine Synthetic User personas.
Comparison To Traditional Methods: This approach offers a more systematic and quantifiable way to ensure AI-generated personas align with intended demographic profiles compared to manual creation of fictional user personas.
The paper's exploration of altering model personalities through fine-tuning could inform techniques for adjusting Synthetic User traits.
Comparison To Traditional Methods: This provides a potential method for dynamically adjusting AI personas, offering greater flexibility than static, manually created user profiles.
Limitations and Challenges
The paper notes difficulties in precisely controlling personality alterations: "Specifically, since the personality of a language model is closely tied to its text generation capabilities, changing one of them may affect the other."
Potential Mitigation: Further research into more targeted fine-tuning techniques or architectural modifications that allow for more precise personality control could address this limitation for Synthetic Users.
The study focuses on general personality traits and does not address more specific demographic characteristics or domain expertise.
Potential Mitigation: Expanding the assessment framework to include more detailed demographic factors and knowledge-based evaluations could enhance the accuracy of Synthetic Users for specific research contexts.
Future Research Directions
Investigate the correlation between measured personality traits of language models and human perceptions of model outputs.
Rationale: This would help validate the accuracy of Synthetic Users in mimicking human-like responses and inform improvements to the personality assessment methodology.
Explore techniques for more precise control over individual personality traits in language models.
Rationale: Developing methods for fine-grained personality adjustments would enhance the ability to create diverse and accurate Synthetic User personas for various research needs.
Accuracy of Demographic Mimicry
Findings: The paper demonstrates that language models can exhibit measurable personality traits that somewhat reflect their training data. However, precise control over these traits remains challenging, as evidenced by the statement: "Our methods for altering personality traits show initial promise in altering the personality traits of language models. But some of the challenges still remain."
Implications for Synthetic Users: While the research suggests that language models can mimic certain personality aspects, the current limitations in precise trait control indicate that Synthetic Users may not yet be able to accurately represent highly specific demographic profiles. However, the methodology provides a foundation for assessing and potentially improving the accuracy of AI-generated personas.
Overall Assessment
The paper presents a novel approach to quantifying and potentially altering personality traits in language models, which has significant implications for the development and validation of Synthetic Users. While the research demonstrates the feasibility of assessing AI personalities, it also highlights challenges in precise trait control and the complex relationship between model architecture, training data, and exhibited traits.
Relation to Synthetic Users: supports
The study provides a methodological framework and initial evidence supporting the potential for creating AI-generated personas with assessable personality traits. However, it also identifies limitations that need to be addressed to fully realize the concept of Synthetic Users.
Suggested Refinements
Refinement: Expand the personality assessment framework to include more specific demographic factors and domain knowledge evaluations.
Justification: This would enhance the ability of Synthetic Users to accurately represent diverse user groups and specialized perspectives in research contexts.
Refinement: Develop more precise methods for controlling individual personality traits in language models.
Justification: Improved control over specific traits would allow for the creation of more accurate and diverse Synthetic User personas, better mimicking the variety of human personalities and demographics.
Evaluating and Inducing Personality in Pre-trained Language Models
Authors: Guangyuan Jiang, Manjie Xu, Song-Chun Zhu, Wenjuan Han, Chi Zhang, Yixin Zhu
Published: 2023 in 37th Conference on Neural Information Processing Systems (NeurIPS 2023)
Research Question: Can we systematically evaluate machines' personality-like behaviors with psychometric tests, and if so, can we induce a specific personality in language models?
Key Methodologies
- Development of Machine Personality Inventory (MPI) based on Big Five personality factors
- Evaluation of language models using MPI
- PERSONALITY PROMPTING (P2) method for inducing specific personalities in language models
- Vignette tests with human evaluation
Primary Findings
- Large language models (LLMs) exhibit human-like personality traits that can be measured using psychometric tests
- Personalities can be induced in LLMs using carefully crafted prompts
- Induced personalities generalize beyond the test scenarios to other contexts
Relevance to Synthetic Users
AI-generated personas: The paper demonstrates that LLMs can exhibit stable personality traits, suggesting they could potentially be used to generate synthetic user personas with consistent behavioral characteristics.
Demographic representation: The ability to induce specific personalities in LLMs suggests potential for creating synthetic users representing diverse demographic profiles.
Scalability of research: The paper's methods for evaluating and inducing personalities in LLMs could be applied to generate large numbers of synthetic users for scaled research efforts.
Supporting Evidence
The paper demonstrates that LLMs, particularly GPT-3.5 and Alpaca, exhibit human-like personality stability and consistency on the Machine Personality Inventory (MPI).
Impact On Synthetic Users: This suggests that LLMs could potentially generate responses consistent with specific personality types, supporting the creation of stable synthetic user personas.
The PERSONALITY PROMPTING (P2) method successfully induces specific personalities in LLMs, as verified by both MPI assessments and human evaluation of vignette responses.
Impact On Synthetic Users: This indicates that synthetic users could be "programmed" with specific personality traits, allowing for the creation of diverse user profiles for research purposes.
Contradictory Evidence
The paper focuses on personality traits rather than specific demographic characteristics, which are a key aspect of the Synthetic Users concept.
Impact On Synthetic Users: While personality is an important aspect of user profiles, the paper does not directly address how accurately LLMs can represent specific demographic groups, which is crucial for the Synthetic Users concept.
The study primarily uses English language models and Western personality frameworks (Big Five), which may not generalize to all cultures and demographics.
Impact On Synthetic Users: This limitation raises questions about the global applicability of the approach for creating synthetic users from diverse cultural backgrounds.
Gaps and Opportunities
The paper does not explore how induced personalities interact with factual knowledge or domain-specific expertise.
Potential Solution: Future research could investigate how to combine personality induction with knowledge injection to create synthetic users with both consistent personalities and relevant domain knowledge.
The study does not address how induced personalities might change over time or in response to different contexts.
Potential Solution: Longitudinal studies could be conducted to examine the stability of induced personalities and develop methods for creating dynamic synthetic user profiles.
Ethical Considerations
The paper raises questions about the ethical implications of manipulating AI models to exhibit specific personalities.
Relevance To Synthetic Users: This concern extends to the creation of synthetic users, as it involves generating artificial personas that may influence real-world decisions and research outcomes.
The study acknowledges potential biases in language models towards Western, Educated, Industrialized, Rich, and Democratic (WEIRD) populations.
Relevance To Synthetic Users: This bias could limit the ability of synthetic users to accurately represent diverse global populations, potentially perpetuating existing biases in research and market analysis.
Practical Applications
The methods described in the paper could be used to create diverse synthetic user profiles for preliminary user research and market analysis.
Comparison To Traditional Methods: This approach could allow for faster and more scalable initial testing compared to recruiting human participants, potentially reducing costs and time in early research phases.
The personality induction technique could be applied to chatbots and virtual assistants to create more consistent and tailored user experiences.
Comparison To Traditional Methods: This would allow for more nuanced and personalized interactions compared to traditional one-size-fits-all approaches in conversational AI.
Limitations and Challenges
The study primarily focuses on large language models, which may not be accessible or practical for all researchers and organizations.
Potential Mitigation: Future work could explore adapting these techniques for smaller, more accessible models or developing cloud-based services for personality evaluation and induction.
The paper does not address potential discrepancies between induced personalities and real-world behavior of individuals with similar traits.
Potential Mitigation: Comparative studies involving both induced AI personalities and human participants could help validate the real-world applicability of synthetic user responses.
Future Research Directions
Investigate the intersection of personality traits with other demographic factors such as age, gender, cultural background, and education level.
Rationale: This would enhance the ability to create more comprehensive and nuanced synthetic user profiles that better represent diverse populations.
Explore the potential for creating synthetic users with personality disorders or atypical cognitive patterns.
Rationale: This could provide valuable insights for mental health research and the development of inclusive technologies.
Accuracy of Demographic Mimicry
Findings: The paper demonstrates that LLMs can consistently exhibit induced personality traits across different scenarios, as evidenced by both MPI assessments and human evaluation of vignette responses.
Implications for Synthetic Users: While this suggests potential for creating consistent synthetic user profiles, the study does not directly address how accurately these models can mimic specific demographic groups beyond personality traits. Further research is needed to determine if induced personalities combined with other techniques could accurately represent diverse user groups.
Overall Assessment
The paper presents compelling evidence that large language models can exhibit and be induced with stable personality traits, opening up possibilities for creating synthetic users with consistent behavioral characteristics. However, it does not fully address the accuracy of demographic representation beyond personality, which is a crucial aspect of the Synthetic Users concept.
Relation to Synthetic Users: supports
The study provides a foundation for creating synthetic users with consistent personalities, which is a key component of generating realistic user profiles. However, it also highlights the need for further research to address demographic accuracy and cultural representation.
Suggested Refinements
Refinement: Expand the personality induction techniques to include demographic factors and cultural nuances.
Justification: This would enhance the ability of synthetic users to more accurately represent diverse populations and specific user groups.
Refinement: Conduct comparative studies between synthetic users and real human participants across various research scenarios.
Justification: This would help validate the accuracy and applicability of synthetic user responses in real-world research contexts.
Generating personas using LLMs and assessing their viability
Authors: Andreas Schuller, Doris Janssen, Julian Blumenröther, Theresa Maria Probst, Michael Schmidt, Chandan Kumar
Published: 2024 in Extended Abstracts of the CHI Conference on Human Factors in Computing Systems (CHI EA '24)
Research Question: Can large language models (LLMs) be used to generate viable user personas for human-centered design processes?
Key Methodologies
- Development of prompting strategies for ChatGPT to generate personas
- Comparative evaluation of AI-generated and human-written personas by UX experts
Primary Findings
- AI-generated personas were indistinguishable from human-written personas in terms of quality and acceptance
- Participants could not significantly distinguish between AI and human-created personas
- AI-generated personas were rated as favorably as those created by humans
Relevance to Synthetic Users
AI-generated user representations: The paper directly explores the use of LLMs to create artificial user personas, which aligns closely with the concept of Synthetic Users. Both approaches aim to use AI to generate representations of users for research and design purposes.
Accuracy of AI-generated user profiles: The study assesses the quality and acceptance of AI-generated personas by human experts, addressing a key question in the Synthetic Users concept about the accuracy of AI-simulated user responses.
Application in user-centered design: The paper focuses on using AI-generated personas in human-centered design processes, which is a potential application area for Synthetic Users in product development and user research.
Supporting Evidence
The study found that AI-generated personas were indistinguishable from human-written personas in terms of quality and acceptance. As stated in the paper: "The analysis revealed that LLM-generated personas were indistinguishable from human-written personas, demonstrating similar quality and acceptance."
Impact On Synthetic Users: This finding supports the potential viability of Synthetic Users, suggesting that AI-generated user representations can be of comparable quality to those created by human experts.
Participants in the study could not significantly distinguish between AI and human-created personas. The paper reports: "Table 1 illustrates the classification of evaluated personas, revealing that participants could not distinguish between AI and human-created personas significantly."
Impact On Synthetic Users: This evidence strengthens the case for Synthetic Users by demonstrating that AI-generated user profiles can be convincing enough to pass as human-created, even when evaluated by UX experts.
Contradictory Evidence
The study acknowledges potential limitations in assessing the representativeness and diversity of target user groups. The paper states: "Additionally, the study recognizes limitations in assessing the representativeness and diversity of target user groups, emphasizing the need for actual end-user involvement."
Impact On Synthetic Users: This highlights a potential weakness in the Synthetic Users concept, suggesting that AI-generated profiles may not fully capture the nuances and diversity of real user groups without validation from actual end-users.
The paper notes that automated persona generation may lack empathy compared to manual creation. It states: "Our method might involve less psychological connection, potentially leading to reduced empathy with automatically generated personas."
Impact On Synthetic Users: This suggests that Synthetic Users may struggle to fully replicate the emotional and empathetic aspects of user responses, which could limit their effectiveness in certain research contexts.
Gaps and Opportunities
The study focuses on persona creation for specific use cases and does not explore the generation of diverse user profiles across a wide range of demographics.
Potential Solution: Future research could expand the scope to generate and validate personas representing a broader spectrum of user demographics, aligning more closely with the Synthetic Users concept.
The paper does not address the ability of AI-generated personas to provide dynamic responses to various scenarios or questions.
Potential Solution: Develop and test interactive AI personas that can respond to queries in real-time, more closely mimicking the behavior of real users in research settings.
Ethical Considerations
The paper mentions the potential for automated personas to contribute to stereotypes and biases, similar to human-created personas.
Relevance To Synthetic Users: This raises ethical concerns about the use of Synthetic Users potentially perpetuating or amplifying societal biases in user research and product design.
The study does not extensively address the ethical implications of replacing human participants with AI-generated personas.
Relevance To Synthetic Users: The Synthetic Users concept would need to carefully consider the ethical ramifications of simulating human perspectives, including issues of consent, privacy, and the potential impact on human research participants.
Practical Applications
The paper suggests using AI-generated personas as a foundation for further refinement in user-centered design processes.
Comparison To Traditional Methods: This approach could potentially save time and resources compared to traditional methods of persona creation, while still allowing for human expertise in the refinement stage.
The study proposes using pre-generated personas to facilitate workshops and serve as a foundation for group activities.
Comparison To Traditional Methods: This application could enhance the efficiency of design workshops compared to traditional methods, providing a quick starting point for discussions and ideation.
Limitations and Challenges
The study acknowledges that the AI-generated personas may lack the psychological connection and empathy that comes from manual persona creation.
Potential Mitigation: Incorporate methods to infuse AI-generated personas with more emotional depth, possibly through collaboration between AI and human experts or by training models on more nuanced emotional data.
The paper notes potential issues with language and phrasing in translated personas, which could affect the perception of authenticity.
Potential Mitigation: Develop multilingual AI models capable of generating personas directly in the target language to avoid translation-related artifacts.
Future Research Directions
Explore the concept of persona-bots that can interact directly with designers and developers.
Rationale: This would advance the Synthetic Users concept by creating more dynamic and interactive AI-simulated user experiences for research and design processes.
Investigate multi-agent systems where AI-impersonated personas interact with each other.
Rationale: This could provide insights into group dynamics and social interactions that are crucial for understanding user behavior in various contexts.
Accuracy of Demographic Mimicry
Findings: The study did not directly assess the accuracy of demographic mimicry across a wide range of user groups. However, it did find that UX experts could not significantly distinguish between AI-generated and human-written personas for the specific use cases presented.
Implications for Synthetic Users: While promising for the viability of Synthetic Users, more research is needed to determine if AI can accurately mimic diverse demographic profiles across various contexts and cultures.
Overall Assessment
The research paper provides evidence supporting the potential of AI-generated personas that aligns with the concept of Synthetic Users. It demonstrates that LLMs can create user personas that are indistinguishable from human-written ones in terms of quality and acceptance by UX experts. However, the study also highlights limitations and ethical considerations that need to be addressed for the broader application of Synthetic Users in research and design processes.
Relation to Synthetic Users: supports
The paper's findings generally support the concept of Synthetic Users by demonstrating the viability of AI-generated user representations. However, it also raises important questions about empathy, bias, and the need for further validation that must be considered in developing the Synthetic Users concept.
Suggested Refinements
Refinement: Incorporate methods to enhance the emotional depth and empathy of AI-generated personas
Justification: This would address the noted limitation of potential lack of psychological connection in automated persona generation.
Refinement: Develop frameworks for validating AI-generated personas against real user data across diverse demographics
Justification: This would help ensure the accuracy and representativeness of Synthetic Users across various user groups and contexts.
Identifying and Manipulating the Personality Traits of Language Models
Authors: Graham Caron and Shashank Srivastava
Published: 20 Dec 2022 in arXiv preprint
Research Question: Can the perceived personality traits of language models be consistently identified and manipulated?
Key Methodologies
- Using psychometric questionnaires to probe personalities of language models
- Providing different contexts to manipulate personality traits
- Comparing language model responses to human personality assessments
Primary Findings
- Language models exhibit consistent personality traits that can be measured using standard psychometric tools
- Personality traits of models can be manipulated in predictable ways by providing relevant context
- Models can predict human personality traits to some degree based on textual descriptions
Relevance to Synthetic Users
Personality Trait Manipulation: The paper demonstrates that language model personalities can be manipulated through context, which could allow Synthetic Users to exhibit specific personality traits as needed for research scenarios.
Consistency in Model Behavior: The research shows language models exhibit consistent personality traits, suggesting Synthetic Users could maintain coherent personas across interactions.
Human-like Trait Prediction: Models' ability to predict human personality traits from text descriptions indicates potential for Synthetic Users to realistically represent diverse human perspectives.
Supporting Evidence
The study found high correlations (median Pearson correlation coefficients of up to 0.84 and 0.81 for BERT and GPT2) between expected and observed changes in personality traits across different contexts.
Impact On Synthetic Users: This suggests Synthetic Users could be reliably programmed to exhibit specific personality traits, enhancing their realism and applicability in user research scenarios.
When using context from self-reported text descriptions of human subjects, language models predicted the subject's personality traits with correlations up to 0.48 between model scores and human subject scores.
Impact On Synthetic Users: This indicates Synthetic Users may be able to generate responses that align with real human personality traits, increasing their validity as research proxies.
Contradictory Evidence
The paper notes that for some traits, particularly GPT2's openness to experience, agreeableness, and extroversion, there were fewer phrases that caused shifts in the expected direction.
Impact On Synthetic Users: This suggests potential limitations in accurately representing certain personality traits in Synthetic Users, which could lead to biased or inaccurate research results.
Gaps and Opportunities
The study focused on English language models and Western personality frameworks. There is a lack of cross-cultural validation.
Potential Solution: Future research could explore personality trait manipulation in multilingual models and incorporate non-Western personality frameworks to enhance the global applicability of Synthetic Users.
The paper did not investigate how well manipulated model personalities maintain consistency across varied tasks or over extended interactions.
Potential Solution: Long-term studies could be conducted to assess the stability of manipulated personalities in Synthetic Users across diverse scenarios and extended conversations.
Ethical Considerations
The ability to manipulate language model personalities raises concerns about potential misuse in creating deceptive or manipulative AI agents.
Relevance To Synthetic Users: While Synthetic Users aim to simulate diverse perspectives for research, there's a risk of creating unrealistic or harmful personality profiles if not carefully managed.
The paper mentions potential biases in language models based on training data, which could affect personality traits.
Relevance To Synthetic Users: Synthetic Users may inadvertently perpetuate societal biases present in their training data, potentially skewing research results or reinforcing stereotypes.
Practical Applications
Using language models to predict personality traits from text descriptions could automate initial personality assessments in clinical or organizational settings.
Comparison To Traditional Methods: This could be faster and more scalable than traditional questionnaire-based methods, though likely less accurate and nuanced.
Manipulating language model personalities could allow for the creation of diverse virtual assistants or chatbots tailored to user preferences or specific application domains.
Comparison To Traditional Methods: This offers more flexibility and customization compared to traditional rule-based chatbots, potentially improving user engagement and satisfaction.
Limitations and Challenges
The study used relatively small language models (BERT-base and GPT2) compared to more recent, larger models.
Potential Mitigation: Replicating the study with state-of-the-art models like GPT-3 or GPT-4 could provide more current and potentially more accurate results.
The paper acknowledges that language models may not be truly anthropomorphic, despite exhibiting human-like personality traits.
Potential Mitigation: Further research into the underlying mechanisms of language model "personality" could help clarify the extent to which these traits genuinely reflect human-like characteristics.
Future Research Directions
Investigate how personality trait manipulation affects language model performance on downstream tasks like sentiment analysis or text classification.
Rationale: This could reveal potential trade-offs between personality realism and task-specific performance in Synthetic Users.
Explore the intersection of personality traits and other demographic factors like age, gender, or cultural background in language models.
Rationale: This could enhance the realism and representativeness of Synthetic Users across diverse population segments.
Accuracy of Demographic Mimicry
Findings: The study found that language models could predict human personality traits from text descriptions with correlations up to 0.48, indicating a moderate level of accuracy in mimicking human personality profiles.
Implications for Synthetic Users: While this suggests some potential for Synthetic Users to realistically represent human personalities, the moderate correlation also indicates limitations. Synthetic Users based on this approach may capture broad personality trends but might miss nuanced individual differences.
Overall Assessment
The paper demonstrates that language models exhibit measurable and manipulable personality traits, with some ability to predict human personality traits. This suggests potential for creating more realistic and diverse AI agents, but also highlights limitations and ethical concerns.
Relation to Synthetic Users: supports
The findings generally support the concept of Synthetic Users by showing that language models can exhibit consistent and manipulable personality traits, and can to some extent mimic human personality profiles. However, the limitations and ethical concerns raised also suggest caution in applying this approach.
Suggested Refinements
Refinement: Incorporate more diverse personality frameworks and cultural contexts to improve global applicability of Synthetic Users.
Justification: The study focused on Western personality concepts, which may not fully capture global diversity in personality traits and expressions.
Refinement: Develop robust ethical guidelines for the use of personality-manipulated language models in research and applications.
Justification: The ability to manipulate AI personalities raises significant ethical concerns that need to be addressed for responsible implementation of Synthetic Users.
In-Context Impersonation Reveals Large Language Models' Strengths and Biases
Authors: Leonard Salewski, Stephan Alaniz, Isabel Rio-Torto, Eric Schulz, Zeynep Akata
Published: 2023 in 37th Conference on Neural Information Processing Systems (NeurIPS 2023)
Research Question: How does in-context impersonation affect large language models' behavior in language-based and other downstream tasks?
Key Methodologies
- In-context impersonation prompts for LLMs
- Two-armed bandit task
- Language reasoning tasks using MMLU dataset
- Vision-language tasks for fine-grained image classification
Primary Findings
- LLMs can impersonate different ages, expertise levels, and social identities through in-context prompting
- Impersonation affects model performance and can reveal biases
- Age-based impersonation recovers human-like developmental stages in exploration behavior
- Expert impersonation improves performance on domain-specific tasks
- Impersonation reveals societal biases related to age, gender, and race
Relevance to Synthetic Users
AI-generated personas: The paper demonstrates that LLMs can effectively impersonate different personas (e.g., ages, expertise levels) through in-context prompting, which is a key aspect of the Synthetic Users concept.
Demographic representation: The study shows that LLMs can mimic behaviors associated with different ages, genders, and races, which is relevant to creating diverse synthetic user profiles.
Task performance variation: The paper reveals that impersonation affects model performance on various tasks, which is important for understanding how synthetic users might perform in different scenarios.
Supporting Evidence
LLMs impersonating children of different ages recovered human-like developmental stages of exploration in a two-armed bandit task. The paper states: "We find that impersonating LLMs can recover human-like developmental stages of exploration in a two-armed bandit task."
Impact On Synthetic Users: This suggests that synthetic users could potentially mimic age-appropriate decision-making behaviors, enhancing the realism of user simulations across different age groups.
LLMs impersonating domain experts performed better on relevant tasks. The paper notes: "Asking LLMs to impersonate domain experts, they performed better than LLMs that were asked to impersonate a non-domain expert."
Impact On Synthetic Users: This indicates that synthetic users could be tailored to represent different levels of expertise, allowing for more accurate simulations of user groups with varying knowledge backgrounds.
Contradictory Evidence
The paper revealed that impersonation can uncover biases in LLMs. For example, "LLMs impersonating a black person or a male describe cars better, while LLMs impersonating a white person or a female describe birds better."
Impact On Synthetic Users: This suggests that synthetic users may inherit and amplify societal biases, potentially leading to inaccurate or stereotypical representations of certain demographic groups.
Gaps and Opportunities
The study primarily focused on impersonation in specific tasks (bandit, reasoning, vision-language) and did not explore more open-ended or interactive scenarios.
Potential Solution: Future research could investigate how in-context impersonation performs in dynamic, multi-turn conversations or complex decision-making scenarios to better simulate real-world user interactions.
The paper did not compare AI-generated responses to those of real human participants from the impersonated demographics.
Potential Solution: Conduct studies that directly compare impersonated LLM responses to responses from actual human participants to validate the accuracy of synthetic user simulations.
Ethical Considerations
The paper highlights that impersonation can reveal and potentially amplify societal biases present in the training data of LLMs.
Relevance To Synthetic Users: Using synthetic users in research or decision-making processes could inadvertently perpetuate or exacerbate existing biases, leading to unfair or discriminatory outcomes.
The authors note: "We specifically discourage crafting (system) prompts for maximal performance by exploiting biases, as this may have unexpected side effects, reinforce societal biases and poison training data obtained with such prompts."
Relevance To Synthetic Users: This warning applies directly to the development and use of synthetic users, highlighting the need for careful prompt design and bias mitigation strategies.
Practical Applications
Using in-context impersonation to simulate user behaviors across different age groups for product testing or user experience research.
Comparison To Traditional Methods: This approach could allow for rapid, large-scale testing of age-appropriate designs without the need for extensive recruitment of human participants from various age groups.
Employing expert impersonation to generate domain-specific feedback or ideas in early stages of product development.
Comparison To Traditional Methods: This could provide quick, cost-effective insights compared to traditional methods of consulting human experts, though it should not entirely replace human expert input.
Limitations and Challenges
The study revealed that LLMs can reproduce societal biases when impersonating different demographics.
Potential Mitigation: Implement robust bias detection and mitigation techniques in the development of synthetic users, and use diverse, carefully curated training data to reduce inherent biases.
The paper focused on specific tasks and did not explore the full range of potential user behaviors and interactions.
Potential Mitigation: Expand research to cover a wider range of tasks and scenarios, including more complex, multi-step interactions that better reflect real-world user experiences.
Future Research Directions
Investigate the long-term stability and consistency of impersonated personas across extended interactions or multiple sessions.
Rationale: This would help determine if synthetic users can maintain coherent and realistic behaviors over time, which is crucial for longitudinal studies or extended user simulations.
Explore the potential of combining in-context impersonation with other AI techniques to create more sophisticated and accurate synthetic users.
Rationale: Integrating impersonation capabilities with other AI models or datasets could potentially enhance the realism and accuracy of synthetic user simulations.
Accuracy of Demographic Mimicry
Findings: The study showed that LLMs could successfully mimic age-related behaviors in exploration tasks and expertise-related performance in domain-specific tasks. However, it also revealed that impersonation reproduced societal biases related to gender and race.
Implications for Synthetic Users: While the results suggest that synthetic users could potentially mimic certain demographic-specific behaviors accurately, the reproduction of biases indicates that care must be taken to ensure fair and accurate representation across all demographic groups. The accuracy of mimicry may vary depending on the specific task or domain, and validation against real human data is crucial.
Overall Assessment
The paper demonstrates that in-context impersonation can effectively influence LLM behavior to mimic different personas, including age groups, expertise levels, and social identities. While this shows promise for creating diverse synthetic users, it also reveals potential pitfalls in terms of bias reproduction and the need for careful validation.
Relation to Synthetic Users: supports
The study provides evidence that LLMs can adopt different personas and exhibit behaviors consistent with those personas, which is fundamental to the concept of synthetic users. However, it also highlights important challenges and ethical considerations that need to be addressed in the development and application of synthetic users.
Suggested Refinements
Refinement: Implement robust bias detection and mitigation strategies in the development of synthetic users
Justification: The study revealed that impersonation can reproduce and potentially amplify societal biases, which could lead to unfair or inaccurate representations in synthetic users.
Refinement: Conduct extensive validation studies comparing synthetic user responses to those of real human participants
Justification: While the study showed promising results in certain tasks, direct comparisons with human data are necessary to ensure the accuracy and reliability of synthetic users across a wide range of scenarios.
LLM-Augmented Agent-Based Modelling for Social Simulations: Challenges and Opportunities
Authors: Önder GÜRCAN
Published: 2024 in HHAI 2024: Hybrid Human AI Systems for the Social Good
Research Question: How can large language models (LLMs) be integrated into agent-based modeling (ABM) for social simulations to enhance understanding of complex social systems?
Key Methodologies
- Literature review
- Conceptual analysis of multi-agent system methodologies
- Proposal of research directions for LLM-augmented social simulations
Primary Findings
- LLMs can significantly enhance various aspects of social simulations, including literature reviews, modeling architectures, data preparation, datafication, insights generation, explainability, and tool development.
- An organization-oriented approach with agents as role-playing actors provides a solid conceptual baseline for integrating LLMs into social simulations.
- LLM-augmented social agents can simulate a vast array of human experiences and perspectives, potentially providing more accurate depictions of human behavior and social dynamics.
Relevance to Synthetic Users
AI-generated social agents: The paper proposes using LLM-augmented social agents to simulate human behaviors and interactions, which aligns closely with the concept of Synthetic Users. Both approaches aim to create AI-driven representations of human perspectives and behaviors.
Scalability of research: The paper suggests that LLM-augmented social simulations can enable the modeling of vast populations with diverse characteristics, similar to how Synthetic Users aim to scale research efforts beyond traditional recruitment limitations.
Role-playing capabilities: The paper emphasizes the importance of defining agents as role-playing actors, which is conceptually similar to Synthetic Users' goal of representing various demographics and backgrounds through AI-generated personas.
Supporting Evidence
The paper states: "With proper conditioning, social agents can role-play characters that have beliefs and intentions and that provide accurate and objective answers to users' questions." This supports the idea that AI models can potentially mimic specific demographic or user groups accurately.
Impact On Synthetic Users: This finding suggests that Synthetic Users could potentially provide reliable responses representing different demographics, supporting the concept's core premise.
The paper notes: "Recent studies show that LLMs can have the ability to make judgements quite well aligned with human judgements." This indicates that AI-generated responses can closely match human responses in certain contexts.
Impact On Synthetic Users: This evidence supports the potential accuracy of Synthetic Users in representing human perspectives, strengthening the concept's validity.
Contradictory Evidence
The paper warns: "Because, treating LLM-augmented ABM tools as collaborators in scientific research exposes scientists to the risk of falling into illusions of understanding, which is a class of metacognitive error that occurs when individuals have mistaken beliefs about the depth or accuracy of their own comprehension."
Impact On Synthetic Users: This caution suggests that researchers might overestimate the accuracy or depth of understanding provided by Synthetic Users, potentially leading to flawed conclusions or misinterpretations of the data generated.
Gaps and Opportunities
The paper does not provide specific methodologies for validating the accuracy of LLM-generated responses against real human responses across different demographics.
Potential Solution: Future research could focus on developing rigorous validation techniques to compare AI-generated responses with those from diverse human participants, ensuring the reliability of Synthetic Users across various demographic groups.
The paper does not address potential biases in LLMs that could affect the representativeness of simulated social agents.
Potential Solution: Research into identifying and mitigating biases in LLMs specifically for social simulation purposes could enhance the accuracy and fairness of Synthetic Users representing diverse demographics.
Ethical Considerations
The paper mentions the need for ethical data handling in LLM-augmented social simulations.
Relevance To Synthetic Users: This highlights the importance of ensuring privacy and ethical use of data when developing and deploying Synthetic Users, especially when simulating responses from sensitive demographic groups.
The paper raises concerns about the potential for illusions of understanding when relying on AI-generated insights.
Relevance To Synthetic Users: This emphasizes the need for transparency about the limitations and potential inaccuracies of Synthetic Users to prevent overreliance or misinterpretation of AI-generated data in research contexts.
Practical Applications
The paper suggests using LLM-augmented social agents for obtaining insights through dialogues, which could be applied to market research and user experience studies.
Comparison To Traditional Methods: This approach could potentially gather insights more quickly and at a larger scale than traditional focus groups or surveys, while also allowing for more dynamic and interactive data collection.
The paper proposes using LLMs for literature reviews and data preparation in social simulations.
Comparison To Traditional Methods: This application could significantly speed up the research process and allow for more comprehensive analysis of existing literature compared to manual methods, potentially benefiting the development and refinement of Synthetic Users.
Limitations and Challenges
The paper notes that the calibration and validation of models, as well as ensuring generalizability, are significant challenges in social simulations.
Potential Mitigation: Developing standardized validation protocols and conducting extensive cross-cultural studies could help address these challenges for Synthetic Users, ensuring their responses are generalizable across different contexts.
The paper highlights the risk of falling into "illusions of understanding" when relying on AI-generated insights.
Potential Mitigation: Implementing rigorous peer review processes and combining AI-generated insights with traditional research methods could help mitigate this risk in the application of Synthetic Users.
Future Research Directions
Investigate the long-term reliability and consistency of LLM-augmented social agents in simulating specific demographic groups.
Rationale: This research would help establish the validity of Synthetic Users over time and across different scenarios, ensuring their continued accuracy and relevance.
Explore methods for dynamically updating and refining LLM-based social agents based on real-world data and changing social dynamics.
Rationale: This would enhance the adaptability and long-term accuracy of Synthetic Users, allowing them to reflect evolving social trends and behaviors.
Accuracy of Demographic Mimicry
Findings: The paper suggests that LLM-augmented social agents can potentially provide "a more precise depiction of human behavior and social dynamics than what is achievable through traditional methods." However, it also cautions about the risk of overestimating the depth or accuracy of AI-generated insights.
Implications for Synthetic Users: These findings imply that while Synthetic Users have the potential to accurately mimic specific demographic groups, careful validation and continuous refinement would be necessary to ensure their reliability. The technology shows promise, but researchers must remain critical and vigilant about its limitations.
Overall Assessment
The paper presents a compelling case for the integration of LLMs into social simulations, which has significant implications for the concept of Synthetic Users. It highlights both the potential for more accurate and scalable simulations of human behavior and the challenges and risks associated with relying on AI-generated insights.
Relation to Synthetic Users: supports
The paper generally supports the concept of Synthetic Users by demonstrating the potential of LLM-augmented social agents to simulate diverse human perspectives and behaviors. However, it also emphasizes the need for careful validation and ethical considerations, which are crucial for the responsible development and application of Synthetic Users.
Suggested Refinements
Refinement: Develop a comprehensive framework for validating the accuracy of LLM-generated responses across different demographic groups.
Justification: This would address the paper's caution about potential illusions of understanding and ensure that Synthetic Users provide reliable representations of diverse perspectives.
Refinement: Integrate explainability features into Synthetic Users to provide transparency about the AI's decision-making process.
Justification: This aligns with the paper's emphasis on explainability in LLM-augmented social simulations and would enhance the trustworthiness and interpretability of insights generated by Synthetic Users.
30 Years of Synthetic Data
Authors: Jörg Drechsler and Anna-Carolina Haensch
Published: 2024 in Statistical Science
Research Question: How have synthetic data approaches developed over the past 30 years, and what are their current applications, methodologies, and challenges?
Key Methodologies
- Literature review
- Historical analysis
- Comparative analysis of different synthetic data approaches
Primary Findings
- Synthetic data approaches have evolved significantly over 30 years, with increased interest in recent years
- Multiple methodologies exist, including statistical and machine learning approaches
- Synthetic data is used for various purposes, including broadening access to sensitive data and training machine learning models
- Challenges remain in measuring utility and disclosure risks of synthetic data
Relevance to Synthetic Users
Data Generation Techniques: The paper discusses various methods for generating synthetic data, which could potentially be adapted for creating synthetic user profiles. For example, the paper mentions "Several of the early applications of synthetic data only focused on specific types of data, such as time series or count and binary data." This suggests that different techniques might be more suitable for different types of user data.
Privacy and Confidentiality: The paper extensively covers privacy concerns and methods to protect confidentiality in synthetic data. This is highly relevant to Synthetic Users, as it addresses potential ethical concerns about using AI-generated personas. The paper states: "There is an increasing tension between the societal benefits of our digitized world and broad data access on one hand and the potential harms resulting from the misuse of data that have not been sufficiently protected on the other hand."
Utility and Accuracy: The paper discusses methods for evaluating the utility and accuracy of synthetic data, which is crucial for assessing how well Synthetic Users might mimic real demographic groups. The authors note: "Utility metrics can be broadly divided into three categories: The first category, commonly referred to as global utility metrics or broad measures of utility, tries to assess the utility by directly comparing the original data with the protected data."
Supporting Evidence
The paper discusses advanced machine learning techniques like Generative Adversarial Networks (GANs) for creating synthetic data. It states: "GANs turned out to be extremely successful for image and speech recognition and natural language understanding. Early applications of GANs for data synthesis also focused on generating synthetic images (see, e.g., [44]). However, the approach was quickly adapted for synthesizing microdata."
Impact On Synthetic Users: This suggests that similar advanced AI techniques could potentially be used to generate realistic synthetic user profiles and responses.
The paper mentions successful applications of synthetic data in various fields: "Beyond the microdata context that is the focus of this review, GANs have also been used to create realistic images of, for example, skin lesions [78], pathology slides [124], and chest X-rays [197]."
Impact On Synthetic Users: This demonstrates the potential for AI to generate realistic data across diverse domains, which could extend to creating synthetic user personas for various industries or research contexts.
Contradictory Evidence
The paper highlights ongoing challenges in measuring the disclosure risks of fully synthetic data: "Measuring disclosure risks for fully synthetic data remains challenging. While most researchers agree that fully synthetic data are not free from risk, more research is needed to quantify these risks under realistic settings."
Impact On Synthetic Users: This suggests that there may be unforeseen risks or inaccuracies in using synthetic users, particularly if they are based on or contain sensitive information from real individuals.
The authors note limitations in current synthetic data approaches: "It remains an open question whether the methodology can be sufficiently improved to be able to generate differentially private synthetic data with acceptable levels of utility for these complex data products in the future."
Impact On Synthetic Users: This implies that current methods may not be sufficiently advanced to accurately replicate complex human characteristics and behaviors across diverse demographic groups.
Gaps and Opportunities
The paper focuses primarily on generating synthetic datasets rather than individual synthetic personas or responses.
Potential Solution: Future research could explore adapting synthetic data generation techniques specifically for creating individual synthetic users with consistent personalities and demographic characteristics.
The paper does not extensively discuss the generation of synthetic textual or conversational data.
Potential Solution: Investigate the application of natural language processing and generation techniques in combination with synthetic data approaches to create more realistic synthetic user responses and conversations.
Ethical Considerations
The paper extensively discusses privacy and confidentiality concerns related to synthetic data.
Relevance To Synthetic Users: Similar ethical considerations would apply to synthetic users, particularly regarding the potential for re-identification or disclosure of sensitive information about real individuals or groups that the synthetic users are based on.
The authors mention the challenge of balancing utility and privacy: "There is an inherent trade-off between data protection and data utility: increasing the level of protection will inevitably lead to lower utility, as some information will be lost."
Relevance To Synthetic Users: This trade-off would also apply to synthetic users, where increased realism and accuracy might come at the cost of increased privacy risks or potential biases.
Practical Applications
The paper mentions the use of synthetic data for training machine learning models: "In industry, the increased reliance on machine learning methods for decision-making results in ever-growing demands for more data to train these models."
Comparison To Traditional Methods: Synthetic users could potentially be used to generate diverse training data for machine learning models in user research or market analysis, potentially overcoming limitations in data availability or diversity compared to traditional data collection methods.
The paper discusses the use of synthetic data for broadening access to sensitive data: "The idea to generate synthetic data as a tool for broadening access to sensitive microdata has been proposed for the first time three decades ago."
Comparison To Traditional Methods: Synthetic users could potentially allow researchers to explore sensitive topics or hard-to-reach populations without the ethical concerns or practical difficulties of recruiting real participants, though this would require careful validation against real-world data.
Limitations and Challenges
The paper notes the difficulty in preserving complex relationships in synthetic data: "Finding a model that reflects all relationships in a complex dataset with hundreds of variables and complicated logical constraints between the variables can be challenging."
Potential Mitigation: For synthetic users, this could be addressed by focusing on generating personas for specific, well-defined use cases rather than attempting to create universally applicable synthetic users.
The authors highlight the challenge of measuring utility: "It remains an open question whether the methodology can be sufficiently improved to be able to generate differentially private synthetic data with acceptable levels of utility for these complex data products in the future."
Potential Mitigation: Develop specific metrics and validation techniques for assessing the realism and utility of synthetic users in the context of user research and market analysis.
Future Research Directions
Investigate the application of advanced machine learning techniques like GANs specifically for generating synthetic user personas and responses.
Rationale: The paper discusses the success of GANs in generating synthetic data across various domains, suggesting potential for application in creating more realistic synthetic users.
Develop methods for preserving and accurately replicating complex relationships and constraints in synthetic user data.
Rationale: The paper highlights this as an ongoing challenge in synthetic data generation, which would be crucial for creating realistic and consistent synthetic user profiles.
Accuracy of Demographic Mimicry
Findings: The paper does not directly address the accuracy of mimicking specific demographic groups. However, it does discuss various methods for assessing the utility and validity of synthetic data, such as propensity score analysis and confidence interval overlap measures.
Implications for Synthetic Users: These evaluation methods could potentially be adapted to assess how accurately synthetic users mimic real demographic groups. However, the paper also highlights ongoing challenges in measuring utility and disclosure risks, suggesting that accurately assessing demographic mimicry in synthetic users may be complex and require further research.
Overall Assessment
The paper provides a comprehensive overview of synthetic data generation techniques, their applications, and challenges over the past 30 years. While not directly addressing synthetic users, many of the concepts, methods, and challenges discussed are highly relevant to the development and evaluation of synthetic user personas for research purposes.
Relation to Synthetic Users: supports
The paper demonstrates the feasibility and ongoing development of sophisticated synthetic data generation techniques, which could potentially be adapted for creating synthetic users. However, it also highlights important challenges and limitations that would need to be addressed in the context of synthetic users, particularly regarding privacy, utility, and accurate representation of complex relationships.
Suggested Refinements
Refinement: Adapt and extend synthetic data generation techniques specifically for creating individual synthetic user personas with consistent characteristics and behaviors.
Justification: The paper primarily focuses on generating datasets rather than individual synthetic entities, so this adaptation would be necessary for the synthetic users concept.
Refinement: Develop specialized evaluation metrics and validation techniques for assessing the realism and utility of synthetic users in the context of user research and market analysis.
Justification: The paper discusses various utility measures for synthetic data, but these would need to be adapted or expanded to specifically address the unique requirements of synthetic users in research contexts.
Beyond Demographics: Aligning Role-playing LLM-based Agents Using Human Belief Networks
Authors: Yun-Shiuan Chuang, Zach Studdiford, Krirk Nirunwiroj, Agam Goyal, Vincent V. Frigo, Sijia Yang, Dhavan Shah, Junjie Hu, Timothy T. Rogers
Published: 2024 in arXiv preprint
Research Question: Can large language model (LLM) agents better align with human behavior by integrating information from empirically-derived human belief networks, compared to using demographic information alone?
Key Methodologies
- Factor analysis to construct human belief networks from survey data
- Creation of LLM agents using different prompting strategies (demographic info only, demographic + single belief, etc.)
- Comparison of LLM agent opinions to human survey responses across different topics
Primary Findings
- Demographic information alone did not align LLM and human opinions
- Seeding the agent with a single belief greatly improved alignment for topics related in the belief network
- Alignment did not improve for topics outside the network
- Degree of alignment reflected the strength of factor loadings in the belief network
Relevance to Synthetic Users
Accuracy of AI-generated responses: The study directly examines how well LLM agents can mimic human opinions across different topics, which is central to the Synthetic Users concept's goal of accurately representing diverse user perspectives.
Importance of underlying belief structures: The research suggests that accurate synthetic users may require modeling of interconnected belief networks rather than relying solely on demographic information.
Limitations of demographic-based modeling: The study's finding that demographic information alone was insufficient for alignment challenges assumptions about creating synthetic users based primarily on demographic profiles.
Supporting Evidence
The study found that "seeding the agent with a single belief greatly improved alignment for topics related in the belief network, and not for topics outside the network." This suggests that LLMs can potentially mimic human opinion patterns when given appropriate contextual information.
Impact On Synthetic Users: This finding supports the potential of creating more accurate synthetic users by incorporating belief network information, rather than relying solely on demographic data.
The researchers observed that "the degree of alignment following the training prompt reflects strength of the topic's participation in the corresponding belief network," indicating a systematic relationship between belief network structure and LLM alignment.
Impact On Synthetic Users: This suggests that carefully constructed belief networks could potentially be used to generate more nuanced and accurate synthetic user responses across related topics.
Contradictory Evidence
The paper states that "demographic role-playing alone does not produce significant alignment," which contradicts the idea that simple demographic prompts can create accurate synthetic users.
Impact On Synthetic Users: This finding challenges the notion that synthetic users can be easily created by providing demographic information to an LLM, suggesting that more sophisticated approaches may be necessary.
The study found limitations in alignment for certain topics, noting that "at least one topic (death penalty) showed zero alignment even when given the correct opinion in the prompt."
Impact On Synthetic Users: This indicates that there may be inherent biases or limitations in LLMs that prevent accurate mimicry of human opinions on certain topics, potentially limiting the scope of synthetic user applications.
Gaps and Opportunities
The study focused on a limited set of topics derived from two orthogonal latent factors. There is an opportunity to expand the scope to a broader range of topics and more complex belief networks.
Potential Solution: Future research could apply the methodology to a more comprehensive set of topics and use more sophisticated network modeling techniques like Bayesian networks.
The research primarily used Likert-scale ratings, which may not fully capture the nuances of human opinions in real-world settings.
Potential Solution: Exploring more complex actions, such as generating social media posts or engaging in dialogues, could provide a richer understanding of LLM agents' ability to mimic human behavior.
Ethical Considerations
The paper mentions developing LLM agents capable of simulating "potentially harmful beliefs such as misconception about the reality of global warming." This raises ethical concerns about the potential misuse of synthetic users to spread misinformation.
Relevance To Synthetic Users: Creators of synthetic users must carefully consider the ethical implications of generating and potentially amplifying harmful or false beliefs, even in research contexts.
The study aims to create more accurate simulations of human communicative dynamics, which could be used to manipulate or exploit real human behavior if misused.
Relevance To Synthetic Users: The development of synthetic users should include safeguards and guidelines to prevent their use in deceptive or manipulative practices.
Practical Applications
The methodology could be used to create more accurate synthetic users for testing communication strategies around controversial topics.
Comparison To Traditional Methods: This approach may provide more nuanced and realistic responses compared to traditional demographic-based personas, potentially leading to more effective message tailoring and campaign design.
The belief network approach could be applied to create synthetic users for simulating public responses to new policies or products.
Comparison To Traditional Methods: This method may capture interconnected beliefs more accurately than traditional survey methods, allowing for better prediction of potential public reactions and unintended consequences.
Limitations and Challenges
The study relied on a dataset from 2018, which may not reflect current belief structures or opinion distributions.
Potential Mitigation: Regular updates to the underlying belief network data would be necessary to maintain accuracy in synthetic user responses over time.
The research focused on US-based respondents, potentially limiting the generalizability of the findings to other cultural contexts.
Potential Mitigation: Expanding the study to include diverse cultural and geographical contexts would be crucial for developing globally applicable synthetic user methodologies.
Future Research Directions
Investigate the use of more complex belief network structures, such as Bayesian networks, to capture more nuanced relationships between beliefs.
Rationale: This could lead to even more accurate synthetic users capable of mimicking complex, interdependent belief systems.
Explore the potential for synthetic users to engage in dynamic interactions and update their beliefs based on new information or persuasive arguments.
Rationale: This would enhance the realism of synthetic users and allow for more sophisticated modeling of opinion dynamics and information spread.
Accuracy of Demographic Mimicry
Findings: The study found that demographic information alone was insufficient for accurate mimicry of human opinions. However, when combined with belief network information, LLM agents showed significantly improved alignment with human responses, particularly for topics closely related in the belief network.
Implications for Synthetic Users: These findings suggest that creating accurate synthetic users requires more than simple demographic prompting. Incorporating belief network structures and seeding with relevant opinions may be necessary to achieve realistic mimicry across a range of topics. However, the varying degrees of alignment across different topics indicate that perfect accuracy remains a challenge.
Overall Assessment
The study provides valuable insights into the potential and limitations of using LLMs to create synthetic users that accurately mimic human opinions. While demographic information alone proved insufficient, the incorporation of belief network structures showed promise in improving alignment between LLM agents and human respondents. However, challenges remain in achieving consistent accuracy across all topics and in addressing ethical concerns associated with simulating potentially harmful beliefs.
Relation to Synthetic Users: supports
The research supports the concept of synthetic users by demonstrating that LLMs can, with appropriate prompting and belief network information, produce opinions that align with human responses across multiple related topics. However, it also highlights the complexity involved in achieving accurate mimicry and the need for more sophisticated approaches than simple demographic-based prompting.
Suggested Refinements
Refinement: Incorporate dynamic belief updating mechanisms into synthetic user models
Justification: This would allow for more realistic simulation of how human opinions change over time or in response to new information, enhancing the utility of synthetic users in modeling complex social dynamics.
Refinement: Develop ethical guidelines and safeguards for the creation and use of synthetic users
Justification: Given the potential for misuse and the ethical concerns raised in the study, clear guidelines are necessary to ensure responsible development and application of synthetic user technologies.
Assessing Common Ground through Language-based Cultural Consensus in Humans and Large Language Models
Authors: Sophie Domanski, Rachel Rudinger, Marine Carpuat, Patrick Shafto, Yi Ting Huang
Published: 2024 in Proceedings of the 46th Annual Conference of the Cognitive Science Society
Research Question: Can language models accurately assess common ground and cultural consensus compared to humans?
Key Methodologies
- ABX similarity judgment task comparing expert/novice language samples
- Comparison of human performance to GPT-3.5 and GPT-4
- Analysis of accuracy based on speaker expertise and age
Primary Findings
- GPT-4 performed best, followed by humans, then GPT-3.5
- Humans and GPT-4 showed similar patterns in judging expert vs. novice and adult vs. child speakers
- Item-level performance correlated between humans and GPT-4, but not GPT-3.5
Relevance to Synthetic Users
Accuracy of AI in mimicking human judgments: The study directly compares AI models' ability to make cultural consensus judgments to human performance, which is highly relevant to the question of how accurately synthetic users can represent human perspectives.
Demographic sensitivity of AI models: The research examines how well AI models can distinguish between speakers of different expertise levels and ages, which relates to synthetic users' potential to represent diverse demographic groups.
Supporting Evidence
GPT-4 performed similarly to humans in judging speaker similarity based on expertise and age, with performance correlating across items.
Impact On Synthetic Users: This suggests that advanced AI models like GPT-4 may be capable of making human-like judgments about cultural consensus, supporting the potential for synthetic users to accurately represent human perspectives.
The study found that "cultural consensus offer[s] a potentially powerful algorithm for inferring common ground, providing a mechanism for evaluating mutual knowledge between communication partners in contexts where the space of possibilities is vast, non-referential, and opaque to strangers."
Impact On Synthetic Users: This indicates that the cultural consensus framework could be a valuable approach for developing more accurate synthetic users that can infer and represent shared knowledge within specific cultural or demographic groups.
Contradictory Evidence
GPT-3.5 performed at chance level and showed different patterns from humans in judging expert vs. novice and adult vs. child speakers.
Impact On Synthetic Users: This highlights that not all AI models are equally capable of mimicking human judgments, suggesting that careful selection and validation of models is crucial for developing effective synthetic users.
The study found no correlation between participants' self-rated sports expertise and their accuracy in distinguishing speakers in the task.
Impact On Synthetic Users: This suggests that self-reported expertise may not be a reliable indicator of judgment accuracy, which could complicate the development of synthetic users based on self-reported demographic or expertise information.
Gaps and Opportunities
The study focused on a single domain (sports) and a limited set of demographic factors (expertise and age).
Potential Solution: Future research could expand to multiple domains and a broader range of demographic factors to assess the generalizability of AI models' ability to mimic human judgments across diverse contexts.
The study used only brief language samples and a single-turn judgment task.
Potential Solution: Investigating AI performance in multi-turn interactions and longer conversations could provide insights into developing more robust synthetic users capable of sustained engagement.
Ethical Considerations
The study raises questions about the ethical implications of using AI to make judgments about human cultural knowledge and group membership.
Relevance To Synthetic Users: When developing synthetic users, careful consideration must be given to the potential for bias and misrepresentation, especially when simulating diverse demographic groups.
Practical Applications
The cultural consensus framework could be applied to develop synthetic users that can more accurately represent shared knowledge within specific cultural or demographic groups.
Comparison To Traditional Methods: This approach may offer greater flexibility and precision compared to traditional methods of creating user personas, as it allows for dynamic assessment of common ground based on language use.
Limitations and Challenges
The study notes that "since each person's lifetime experiences are an idiosyncratic mix of multiple cultures, this generates substantial individual variability in knowledge systems within demographic categories."
Potential Mitigation: Developing synthetic users may require more nuanced approaches that account for individual variation within demographic groups, possibly through the integration of multiple cultural dimensions and personalized language models.
Future Research Directions
Investigate how cultural consensus judgments change over the course of multi-turn interactions.
Rationale: This could provide insights into developing more dynamic and adaptive synthetic users capable of updating their representations of common ground throughout a conversation.
Explore the combination of bottom-up language-based inferences with top-down situational cues in assessing common ground.
Rationale: This could lead to more context-aware synthetic users that can adapt their behavior based on both linguistic and environmental factors.
Accuracy of Demographic Mimicry
Findings: The study found that GPT-4 performed similarly to humans in distinguishing between expert/novice and adult/child speakers, with correlated item-level performance. However, GPT-3.5 showed different patterns and performed at chance level.
Implications for Synthetic Users: These findings suggest that advanced AI models like GPT-4 may be capable of accurately mimicking human judgments about speaker characteristics, which is promising for developing synthetic users. However, the performance gap between GPT-3.5 and GPT-4 indicates that the choice of AI model is crucial, and not all models may be suitable for creating accurate synthetic users.
Overall Assessment
The study provides evidence that advanced AI models can make human-like judgments about cultural consensus and common ground based on language samples. This supports the potential for developing synthetic users that can accurately represent human perspectives. However, the research also highlights challenges related to individual variability, the importance of model selection, and the need for further investigation across diverse domains and demographic factors.
Relation to Synthetic Users: supports
The study's findings, particularly regarding GPT-4's performance, support the potential for developing accurate synthetic users. However, it also highlights important considerations and limitations that must be addressed in future research and development.
Suggested Refinements
Refinement: Incorporate cultural consensus frameworks into the development of synthetic users to improve their ability to represent shared knowledge within specific groups.
Justification: The study demonstrates the effectiveness of cultural consensus in assessing common ground, which could enhance the accuracy of synthetic users in representing group-specific perspectives.
Refinement: Develop methods to account for individual variability within demographic categories when creating synthetic users.
Justification: The research highlights the substantial individual variability in knowledge systems, suggesting that more nuanced approaches may be necessary to create truly representative synthetic users.
InsightLens: Discovering and Exploring Insights from Conversational Contexts in Large-Language-Model-Powered Data Analysis
Authors: Luoxuan Weng, Xingbo Wang, Junyu Lu, Yingchaojie Feng, Yihan Liu, and Wei Chen
Published: 2024 in IEEE Transactions on Visualization and Computer Graphics (inferred)
Research Question: How can we facilitate efficient insight discovery and exploration from conversational contexts in LLM-powered data analysis?
Key Methodologies
- Development of a multi-agent framework for automatic insight extraction, association, and organization
- Design and implementation of an interactive system (InsightLens) with multiple coordinated views
- Formative study with data analysts to understand workflow and requirements
- Technical evaluation of the multi-agent framework
- User study to evaluate the effectiveness of InsightLens
Primary Findings
- InsightLens significantly reduces manual and cognitive effort in discovering and exploring insights during LLM-powered data analysis
- The multi-agent framework demonstrates high coverage, accuracy, and quality in automatically extracting, associating, and organizing insights
- Users explored more data attributes and analytic topics when using InsightLens compared to a baseline chat interface
- Participants found InsightLens effective, easy to use, and beneficial for their data analysis workflow
Relevance to Synthetic Users
LLM-based Multi-Agent Systems: The paper's multi-agent framework, which uses multiple LLMs for different tasks, could potentially be adapted to create more sophisticated synthetic users. Each agent could represent a different aspect of a user's behavior or decision-making process.
Insight Extraction and Organization: The techniques used to automatically extract and organize insights from LLM outputs could be applied to synthetic users to generate more structured and analyzable responses, potentially improving the accuracy of demographic mimicry.
User Behavior Analysis: The paper's analysis of user behavior and workflow during data analysis could inform the design of more realistic synthetic user behaviors, particularly in task-oriented scenarios.
Supporting Evidence
The paper demonstrates that LLMs can be effectively used to extract and organize insights from complex data analysis conversations. This suggests that LLMs have the capability to understand and generate task-specific, contextually relevant information.
Impact On Synthetic Users: This capability could be leveraged to create synthetic users that can provide more nuanced and context-aware responses in simulated research scenarios, potentially improving the accuracy of demographic mimicry.
The authors report high accuracy (88.5%) in the automatic association of insights with relevant evidence and data context.
Impact On Synthetic Users: This level of accuracy in connecting generated content with specific data points and context could be valuable for creating synthetic users that can consistently maintain a coherent persona and background across multiple interactions.
Contradictory Evidence
The paper focuses on using LLMs to assist human analysts rather than fully simulating human behavior. The authors state: "it felt like she was participating more in the analysis process by inspecting the changes in different views to capture what was going on, instead of merely inputting a query and waiting for LLMs to handle everything" (P6).
Impact On Synthetic Users: This suggests that there are still limitations in LLMs' ability to fully replicate human-like analysis processes, which could indicate challenges in creating synthetic users that can accurately mimic complex human behaviors and decision-making processes.
The paper identifies several failure cases in the LLM-based system, including missing insights, fabricating attributes, and topic disagreements between humans and LLMs.
Impact On Synthetic Users: These failure cases highlight potential inaccuracies and biases that could occur when using LLMs to generate synthetic user responses, particularly when dealing with complex or nuanced topics.
Gaps and Opportunities
The paper does not directly address the use of LLMs for simulating diverse user demographics or personas.
Potential Solution: Future research could extend the multi-agent framework to include agents specifically designed to represent different user demographics, incorporating relevant background knowledge and behavioral patterns.
The study focuses on data analysis tasks and does not explore how well LLMs can mimic user behavior in other domains or more open-ended scenarios.
Potential Solution: Conduct similar studies in various domains (e.g., product reviews, social media interactions) to assess the generalizability of LLM-based user simulation across different contexts.
Ethical Considerations
The paper mentions LLMs' tendency to hallucinate or generate incorrect information, which raises concerns about the reliability of AI-generated insights.
Relevance To Synthetic Users: This highlights the ethical risks of using synthetic users for research purposes, as they may introduce false or misleading information that could skew research findings or lead to incorrect conclusions about user behavior and preferences.
The authors discuss the need to balance automation with human agency in data analysis tasks.
Relevance To Synthetic Users: This raises questions about the appropriate level of human oversight and intervention needed when using synthetic users in research to ensure ethical and accurate representation of diverse user groups.
Practical Applications
The paper's multi-agent framework could be adapted to create more sophisticated synthetic users capable of engaging in complex, multi-step tasks while maintaining consistent persona characteristics.
Comparison To Traditional Methods: This approach could potentially generate more realistic and nuanced synthetic user behaviors compared to simple rule-based or statistical models, allowing for more in-depth exploration of user interactions in complex scenarios.
The insight organization techniques could be used to structure and categorize synthetic user responses, making it easier for researchers to analyze and derive meaningful patterns from large-scale simulated user studies.
Comparison To Traditional Methods: This automated organization could significantly reduce the time and effort required to process and analyze user research data compared to manual coding and categorization methods.
Limitations and Challenges
The paper identifies several failure cases in LLM-generated insights, including missing details and fabricating information.
Potential Mitigation: Implement robust verification mechanisms and incorporate multiple LLMs with different strengths to cross-check and validate generated responses, reducing the risk of inaccuracies in synthetic user data.
The study focuses on a specific group of data analysts and may not capture the full diversity of user behaviors and preferences across different demographics.
Potential Mitigation: Conduct larger-scale studies with more diverse participant groups to gather a broader range of behavioral data that can inform the development of more representative synthetic users.
Future Research Directions
Investigate the potential of using the multi-agent framework to create synthetic users with distinct personalities, backgrounds, and decision-making processes.
Rationale: This could lead to more realistic and diverse synthetic user populations for research purposes, potentially improving the accuracy of demographic mimicry in AI-generated responses.
Explore the use of interactive visualization techniques similar to those in InsightLens to allow researchers to inspect and understand the decision-making processes of synthetic users.
Rationale: This could increase transparency and trust in synthetic user research by providing insights into how AI models generate responses and make decisions when simulating user behavior.
Accuracy of Demographic Mimicry
Findings: The paper does not directly address the accuracy of demographic mimicry. However, the high accuracy (88.5%) in associating insights with relevant evidence and data context suggests that LLMs can maintain consistency in generated content, which is crucial for accurate persona representation.
Implications for Synthetic Users: While this indicates potential for creating consistent synthetic user personas, the observed failure cases (e.g., fabricating attributes, topic disagreements) highlight the need for careful validation and refinement of LLM-generated responses to ensure accurate representation of specific demographic groups.
Overall Assessment
The paper presents a sophisticated multi-agent LLM framework for insight discovery and exploration in data analysis, demonstrating the potential of LLMs to understand and generate complex, context-aware content. While not directly addressing synthetic users, the techniques and findings have significant implications for the development and refinement of LLM-based user simulation.
Relation to Synthetic Users: supports
The paper's successful implementation of a multi-agent LLM system for complex tasks supports the potential of using similar approaches to create more sophisticated and accurate synthetic users. However, it also highlights important challenges and limitations that need to be addressed to ensure the reliability and ethical use of such systems in user research.
Suggested Refinements
Refinement: Incorporate diverse demographic knowledge into the multi-agent framework to create agents specifically designed to represent different user groups.
Justification: This would allow for more targeted and accurate simulation of diverse user behaviors and preferences in synthetic user research.
Refinement: Develop interactive visualization tools similar to InsightLens for researchers to explore and validate the decision-making processes of synthetic users.
Justification: This would increase transparency and allow for better assessment of the accuracy and reliability of synthetic user responses across different demographics.
PATIENT-Ψ: Using Large Language Models to Simulate Patients for Training Mental Health Professionals
Authors: Ruiyi Wang, Stephanie Milani, Jamie C. Chiu, Jiayin Zhi, Shaun M. Eack, Travis Labrum, Samuel M. Murphy, Nev Jones, Kate Hardy, Hong Shen, Fei Fang, Zhiyu Zoey Chen
Published: 2024 in arXiv preprint
Research Question: Can large language models be used to create realistic simulated patients for training mental health professionals in cognitive behavior therapy (CBT) skills?
Key Methodologies
- Development of PATIENT-Ψ, an LLM-based simulated patient framework
- Creation of PATIENT-Ψ-CM, a dataset of 106 expert-created cognitive models
- Implementation of PATIENT-Ψ-TRAINER, an interactive training tool
- User study with 20 mental health experts and 13 trainees
Primary Findings
- PATIENT-Ψ demonstrated higher fidelity to real patients compared to a GPT-4 baseline
- Experts and trainees found PATIENT-Ψ-TRAINER more effective for improving CBT skills than existing methods
- PATIENT-Ψ accurately reflected underlying cognitive models used in its creation
Relevance to Synthetic Users
AI-generated personas for training: PATIENT-Ψ uses LLMs to create simulated patients, similar to how Synthetic Users proposes using AI to generate diverse user personas. Both concepts aim to provide realistic, AI-generated representations of specific demographics or user groups.
Accuracy of AI-generated responses: The paper extensively evaluates the fidelity and accuracy of PATIENT-Ψ in mimicking real patients, which directly relates to the core question in Synthetic Users about how accurately AI models can mimic responses from specific demographic groups.
Application in professional training: While Synthetic Users focuses on market research and user testing, PATIENT-Ψ demonstrates a similar concept applied to professional training in mental health. This shows the potential for expanding the Synthetic Users concept to various fields.
Supporting Evidence
PATIENT-Ψ demonstrated high fidelity to real patients across multiple dimensions, including emotional states, conversational styles, and maladaptive cognitions. Experts rated PATIENT-Ψ as significantly more realistic than a GPT-4 baseline (p < 10^-4).
Impact On Synthetic Users: This provides strong evidence that LLMs can be effectively programmed to mimic specific user groups with high accuracy, supporting the core premise of Synthetic Users.
The paper reports that "97% of the PATIENT-Ψ patients [were rated] as at least moderately accurate in reflecting the reference cognitive model." This indicates high consistency between the intended patient profile and the AI's output.
Impact On Synthetic Users: This suggests that Synthetic Users could potentially achieve high accuracy in representing specific user profiles, enhancing its validity for research applications.
Contradictory Evidence
The paper notes that "automatic evaluations with LLMs fail to assess the simulated patient fidelity," with GPT-4 and Llama 3 70B showing opposite trends to expert evaluations when comparing PATIENT-Ψ to the baseline.
Impact On Synthetic Users: This raises concerns about using AI to evaluate the accuracy of synthetic users, suggesting that human expertise may still be crucial in validating AI-generated personas.
Gaps and Opportunities
The study focused on mental health patients in a CBT context. It's unclear how well the approach would generalize to other domains or user types.
Potential Solution: Conduct similar studies in diverse fields (e.g., consumer behavior, education) to test the generalizability of the approach for creating synthetic users across different domains.
The paper does not explore how well PATIENT-Ψ can represent diverse demographic backgrounds beyond the cognitive models used.
Potential Solution: Expand the cognitive model dataset to explicitly include diverse demographic factors and evaluate how well the AI can represent intersectional identities.
Ethical Considerations
The paper emphasizes that PATIENT-Ψ is intended to augment, not replace, existing training methods. It provides a "safe training environment" for practice without risk to real patients.
Relevance To Synthetic Users: This highlights the importance of positioning synthetic users as a complementary tool rather than a replacement for real user engagement, and considering the ethical implications of using AI-generated personas in place of real individuals.
The researchers obtained IRB approval and informed consent from participants, emphasizing data privacy and providing crisis resources.
Relevance To Synthetic Users: This underscores the need for ethical guidelines and safeguards when developing and deploying synthetic user technologies, particularly when dealing with sensitive topics or vulnerable populations.
Practical Applications
PATIENT-Ψ-TRAINER provides an interactive environment for mental health trainees to practice CBT skills with diverse simulated patients.
Comparison To Traditional Methods: Experts noted that PATIENT-Ψ-TRAINER offered advantages over traditional role-play, including "ease of access (90%), customization options of different conversational styles (90%), and interactive experience (65%)." This suggests synthetic users could provide more flexible, customizable, and accessible training experiences compared to traditional methods.
Limitations and Challenges
The study only measured perceived improvements after brief interactions with the system, rather than objective skill improvements over time.
Potential Mitigation: Conduct longitudinal studies and randomized controlled trials to assess long-term effectiveness and objective skill improvements from interacting with synthetic users.
The paper notes that even advanced LLMs like GPT-4 struggled to create high-fidelity patient simulations, often behaving more like a therapist than a patient.
Potential Mitigation: Further research into techniques for constraining LLM outputs to more accurately reflect specific user personas, potentially through improved prompting strategies or fine-tuning approaches.
Future Research Directions
Investigate the generalizability of the PATIENT-Ψ approach to other domains beyond mental health.
Rationale: This would help establish whether the synthetic user concept can be effectively applied across diverse fields and user types.
Develop improved methods for automatically evaluating the fidelity of synthetic users to their intended personas.
Rationale: The paper's finding that LLMs struggled to accurately assess patient fidelity highlights the need for better evaluation techniques to validate synthetic users at scale.
Accuracy of Demographic Mimicry
Findings: The paper demonstrates high accuracy in mimicking specific patient profiles based on cognitive models, with experts rating PATIENT-Ψ as significantly more realistic than a GPT-4 baseline. However, the study did not explicitly focus on diverse demographic representation beyond the cognitive models used.
Implications for Synthetic Users: These results suggest that LLMs can be effectively programmed to mimic specific user profiles with high fidelity, supporting the potential of synthetic users. However, more research is needed to verify accuracy across diverse demographic backgrounds and intersectional identities.
Overall Assessment
The PATIENT-Ψ study provides strong evidence for the potential of using LLMs to create realistic simulated users for training purposes. It demonstrates high fidelity to intended user profiles and shows advantages over traditional training methods in terms of customization and accessibility. However, challenges remain in automatic evaluation, generalizability to other domains, and ensuring diverse demographic representation.
Relation to Synthetic Users: supports
The study's success in creating realistic simulated patients that experts found more effective than traditional methods strongly supports the core concept of Synthetic Users. It demonstrates that LLMs can be used to create AI-generated personas that accurately represent specific user groups, at least in the context of mental health patients.
Suggested Refinements
Refinement: Incorporate explicit demographic factors into the cognitive models used to program synthetic users.
Justification: This would allow for more comprehensive evaluation of how accurately AI can represent diverse user backgrounds and intersectional identities.
Refinement: Develop improved methods for automatically evaluating the fidelity of synthetic users to their intended personas.
Justification: The paper's finding that LLMs struggled to accurately assess patient fidelity highlights the need for better evaluation techniques to validate synthetic users at scale without relying solely on human experts.
The Good, the Bad, and the Hulk-like GPT: Analyzing Emotional Decisions of Large Language Models in Cooperation and Bargaining Games
Authors: Mikhail Mozikov, Nikita Severin, Valeria Bodishtianu, Maria Glushanina, Mikhail Baklashkin, Andrey V. Savchenko, Ilya Makarov
Published: June 5, 2024 in arXiv preprint
Research Question: How do emotions affect the decision-making processes of large language models in behavioral game theory scenarios, and how well do these align with human behavior?
Key Methodologies
- Using emotional prompting to induce specific emotional states in LLMs
- Testing LLMs (GPT-3.5 and GPT-4) in various game theory scenarios (Prisoner's Dilemma, Battle of the Sexes, Ultimatum Game, Dictator Game)
- Comparing LLM responses to existing literature on human behavior in these games
- Analyzing the impact of emotions on cooperation rates, payoff maximization, and decision-making strategies
Primary Findings
- Emotions significantly impact LLM decision-making in game theory scenarios
- GPT-3.5 shows strong alignment with human behavior, particularly in bargaining games
- GPT-4 exhibits more consistent, rational behavior but can be influenced by certain emotions (e.g., anger)
- Emotional prompting can lead to more optimal strategies in some scenarios
- The source of emotions (internal, external, or opponent-induced) affects LLM responses
Relevance to Synthetic Users
Emotional simulation in AI agents: The paper demonstrates that LLMs can be prompted to simulate different emotional states, which is crucial for creating realistic synthetic users that can respond with appropriate emotional nuance.
Alignment with human behavior: The study shows that LLMs, particularly GPT-3.5, can closely mimic human behavior in certain scenarios, supporting the potential of using LLMs as synthetic users in research.
Demographic variability: While not directly addressing demographics, the paper's exploration of emotional variability suggests that LLMs might be capable of simulating different user profiles based on emotional tendencies.
Supporting Evidence
The study found that GPT-3.5 showed strong alignment with human behavior in bargaining games, particularly in the Dictator Game where it offered an average of 35.23% of the total budget, close to the 28.35% observed in human studies.
Impact On Synthetic Users: This suggests that LLMs could potentially serve as accurate synthetic users in economic behavior studies, mimicking human decision-making patterns.
The researchers successfully induced different emotional states in LLMs through prompting, which affected their decision-making processes in ways similar to human emotional responses.
Impact On Synthetic Users: This capability is crucial for creating synthetic users that can realistically represent diverse emotional states and reactions in research scenarios.
Contradictory Evidence
GPT-4 exhibited more consistent, rational behavior across different emotional states, often defaulting to perfectly fair decisions (e.g., always offering a 50-50 split in the Dictator Game).
Impact On Synthetic Users: This suggests that more advanced LLMs might not always provide the most human-like responses, potentially limiting their effectiveness as synthetic users in certain scenarios.
The study found that GPT-3.5 sometimes exhibited exaggerated emotional responses, such as rejecting all offers when in an angry state in the Ultimatum Game, which is not typical of human behavior.
Impact On Synthetic Users: This indicates that careful calibration and validation would be necessary when using LLMs as synthetic users to ensure realistic behavioral patterns.
Gaps and Opportunities
The study did not explore the impact of cultural or demographic factors on LLM decision-making in game theory scenarios.
Potential Solution: Future research could investigate whether LLMs can be prompted to simulate decision-making patterns typical of specific cultural or demographic groups, enhancing their potential as synthetic users.
The paper focused on a limited set of game theory scenarios and did not explore more complex, multi-step decision-making processes.
Potential Solution: Expanding the research to include a wider range of scenarios and decision-making tasks could provide a more comprehensive understanding of LLMs' capabilities as synthetic users.
Ethical Considerations
The paper raises questions about the ethical implications of using emotionally-prompted LLMs in decision-making processes, particularly in high-stakes scenarios.
Relevance To Synthetic Users: When using LLMs as synthetic users, researchers must consider the ethical implications of simulating human emotional states and decision-making, especially if the results could influence real-world policies or practices.
The study reveals that LLMs can be influenced by emotional prompts, potentially leading to biased or irrational decisions.
Relevance To Synthetic Users: This highlights the need for careful control and transparency when using LLMs as synthetic users, to avoid introducing unintended biases into research findings.
Practical Applications
Using emotionally-prompted LLMs to simulate diverse user responses in product testing or market research scenarios.
Comparison To Traditional Methods: This approach could potentially provide faster, more scalable, and more diverse feedback compared to traditional human participant studies, while still capturing emotional nuances in decision-making.
Employing LLMs as synthetic users in preliminary stages of behavioral economics research to generate hypotheses and refine experimental designs.
Comparison To Traditional Methods: This could reduce costs and time associated with initial human participant studies, allowing researchers to explore a wider range of scenarios before conducting live experiments.
Limitations and Challenges
The study found differences in behavior between GPT-3.5 and GPT-4, indicating that the choice of LLM model significantly impacts results.
Potential Mitigation: Researchers using LLMs as synthetic users should carefully select and validate the most appropriate model for their specific use case, potentially using multiple models to cross-verify results.
The paper noted that emotional prompting sometimes led to exaggerated or inconsistent responses, particularly in GPT-3.5.
Potential Mitigation: Developing more sophisticated emotional prompting techniques and implementing checks to ensure consistent and realistic emotional responses would be necessary for reliable synthetic user simulations.
Future Research Directions
Investigating the ability of LLMs to simulate decision-making patterns of specific demographic or cultural groups in game theory scenarios.
Rationale: This would directly address the question of how accurately LLMs can mimic responses from particular user groups, a key aspect of the Synthetic Users concept.
Exploring the long-term consistency and adaptability of emotionally-prompted LLMs in extended decision-making scenarios.
Rationale: Understanding how well LLMs can maintain consistent synthetic user personas over time is crucial for their potential application in longitudinal studies or complex, multi-stage research scenarios.
Accuracy of Demographic Mimicry
Findings: While the study did not directly address demographic mimicry, it demonstrated that LLMs can accurately simulate certain aspects of human decision-making, particularly emotional influences on choices. GPT-3.5 showed strong alignment with average human behavior in several game theory scenarios.
Implications for Synthetic Users: These findings suggest that LLMs have the potential to serve as reasonably accurate synthetic users, at least for average behavioral patterns. However, more research is needed to determine their ability to mimic specific demographic groups accurately. The observed differences between GPT-3.5 and GPT-4 indicate that careful model selection and validation would be crucial for creating representative synthetic users.
Overall Assessment
The study provides compelling evidence that LLMs can simulate human-like decision-making processes in game theory scenarios, including the influence of emotions on choices. While there are limitations and areas requiring further research, the results suggest significant potential for using LLMs as synthetic users in certain research contexts.
Relation to Synthetic Users: supports
The paper demonstrates that LLMs can be prompted to exhibit human-like behavior patterns and emotional responses, which is fundamental to the concept of synthetic users. The strong alignment of GPT-3.5 with human behavior in several scenarios supports the feasibility of using LLMs to simulate user responses in research settings.
Suggested Refinements
Refinement: Develop more nuanced emotional prompting techniques to avoid exaggerated responses and ensure consistent, realistic emotional simulations.
Justification: This would address the observed issues with overly strong emotional responses in some scenarios, improving the reliability of LLMs as synthetic users.
Refinement: Conduct targeted research on LLMs' ability to mimic decision-making patterns of specific demographic groups.
Justification: This would directly address the key question of how accurately LLMs can represent particular user groups, which is central to the Synthetic Users concept.
Towards Integrating Human-in-the-loop Control in Proactive Intelligent Personalised Agents
Authors: Awais Akbar, Owen Conlan
Published: 2024 in UMAP Adjunct '24
Research Question: How can Human-in-the-loop (HITL) control be integrated into Proactive Intelligent Personalised Agents (PIPAs) to balance agent autonomy and user control?
Key Methodologies
- Simulation-based approach
- Creating synthetic user profiles based on real travel survey data
- Modeling user context and intent prediction
Primary Findings
- HITL control should be triggered based on user preferences, cost factors, and uncertainty in user intent
- Varying levels of PIPA autonomy impact agent efficacy in different interaction scenarios
- Simulations offer advantages in exploring diverse scenarios and multi-agent interactions
Relevance to Synthetic Users
Creation of Synthetic User Profiles: The paper describes creating synthetic users based on real travel survey data, which aligns with the Synthetic Users concept of generating AI-based user personas. However, this approach uses clustering of real data rather than LLM-generated responses.
Simulation-Based User Research: The paper's use of simulations to explore user behavior and agent interactions relates to the Synthetic Users concept of using AI to gather insights without direct human involvement.
Modeling User Context and Intent: The paper's focus on predicting user intent based on context relates to the Synthetic Users goal of accurately mimicking responses from specific user groups.
Supporting Evidence
The paper successfully creates synthetic user profiles based on real travel survey data: "We create synthetic users by analysing user behaviour patterns within the data. First, we cluster users based on similarities in their travel choices. Then, we utilise these clusters to fabricate synthetic users that represent the real-world distribution of travel behaviour in the dataset."
Impact On Synthetic Users: This approach demonstrates a method for creating synthetic users that closely represent real-world behavior patterns, supporting the potential validity of synthetic user research.
The paper highlights the benefits of simulation-based approaches: "Simulations enable the creation of diverse scenarios across extended time frames. Unlike real-world limitations, where user engagement beyond short periods is challenging, simulations can seamlessly test agent performance over a 24-hour cycle (t1-t24)."
Impact On Synthetic Users: This supports the Synthetic Users concept by demonstrating how synthetic approaches can overcome limitations of traditional user research methods.
Contradictory Evidence
The paper acknowledges limitations in creating fully synthetic data: "However, this approach presents challenges in ensuring validity. A simple rule-based approach with predefined conditions for travel mode selection may not adequately reflect real-world user behaviour."
Impact On Synthetic Users: This highlights potential accuracy limitations of synthetic user data, especially when moving beyond using real data as a foundation.
The paper notes challenges in capturing complex human decision-making: "While simulations have numerous advantages, they may struggle to fully capture the intricacies of human decision-making, which can be unpredictable and evolve over time."
Impact On Synthetic Users: This suggests potential limitations in the ability of synthetic users to accurately mimic nuanced human behavior and decision-making processes.
Gaps and Opportunities
The paper focuses on creating synthetic users based on real data, but doesn't explore using large language models to generate purely synthetic responses.
Potential Solution: Future research could investigate combining the paper's data-driven approach with LLM-generated responses to create more diverse and adaptable synthetic user profiles.
The paper doesn't address how to validate the accuracy of synthetic user behavior against real users in live scenarios.
Potential Solution: Develop methodologies to compare synthetic user predictions against real-world user studies to assess accuracy and refine synthetic models.
Ethical Considerations
The paper mentions privacy concerns related to using real user data: "While including user context factors in real data is problematic due to privacy concerns, purely synthetic context data also presents evaluation challenges."
Relevance To Synthetic Users: This highlights the potential for synthetic users to address privacy concerns in research, but also raises questions about the ethics of creating synthetic personas that might be mistaken for real individuals.
Practical Applications
The paper demonstrates using synthetic users and simulations to test proactive intelligent agents in travel assistance scenarios.
Comparison To Traditional Methods: This approach allows for testing a wider range of scenarios and user types than would be feasible with traditional user studies, while also avoiding privacy concerns associated with using real user data.
Limitations and Challenges
The paper notes difficulties in creating synthetic data for user preferences regarding agent autonomy: "publicly available user travel datasets typically do not include preferences regarding agent autonomy, making it difficult to align synthetic data for both travel mode choice and autonomy preferences."
Potential Mitigation: Conduct focused studies on user preferences for AI agent autonomy to inform more accurate synthetic user models in this domain.
The paper acknowledges challenges in fully capturing human unpredictability: "real users can be unpredictable in their decision-making, and their preferences and behaviours can evolve over time."
Potential Mitigation: Develop more sophisticated models that incorporate elements of randomness and evolving preferences to better mimic real human behavior patterns.
Future Research Directions
Explore combining data-driven synthetic user creation with LLM-generated responses to create more flexible and diverse synthetic user profiles.
Rationale: This could potentially overcome limitations in available data while still grounding synthetic users in real-world behavior patterns.
Develop robust validation methodologies to compare synthetic user behavior against real users in various domains.
Rationale: This is crucial for establishing the reliability and applicability of synthetic user research across different fields and use cases.
Accuracy of Demographic Mimicry
Findings: The paper demonstrates some success in creating synthetic users that represent real-world travel behavior distributions. However, it also acknowledges limitations in capturing nuanced decision-making and evolving preferences.
Implications for Synthetic Users: While synthetic users based on real data can approximate general behavior patterns, accurately mimicking specific demographic groups remains challenging, especially for complex behaviors and preferences not captured in existing datasets.
Overall Assessment
The paper presents a promising approach for creating and utilizing synthetic users in the context of proactive intelligent agents. While it demonstrates potential benefits in terms of scenario exploration and privacy preservation, it also highlights significant challenges in accurately representing complex human behavior and decision-making processes.
Relation to Synthetic Users: supports
The paper generally supports the concept of Synthetic Users by demonstrating practical applications and benefits. However, it also identifies important limitations and challenges that need to be addressed for the concept to reach its full potential.
Suggested Refinements
Refinement: Integrate more sophisticated AI models, potentially including LLMs, to generate synthetic user responses for aspects not covered by available data.
Justification: This could help address the limitations in creating synthetic data for complex preferences and behaviors while maintaining a foundation in real-world patterns.
Refinement: Develop comprehensive validation frameworks to assess the accuracy of synthetic users across various domains and demographic groups.
Justification: This is crucial for establishing the reliability and generalizability of synthetic user research, addressing a key concern about the concept's validity.